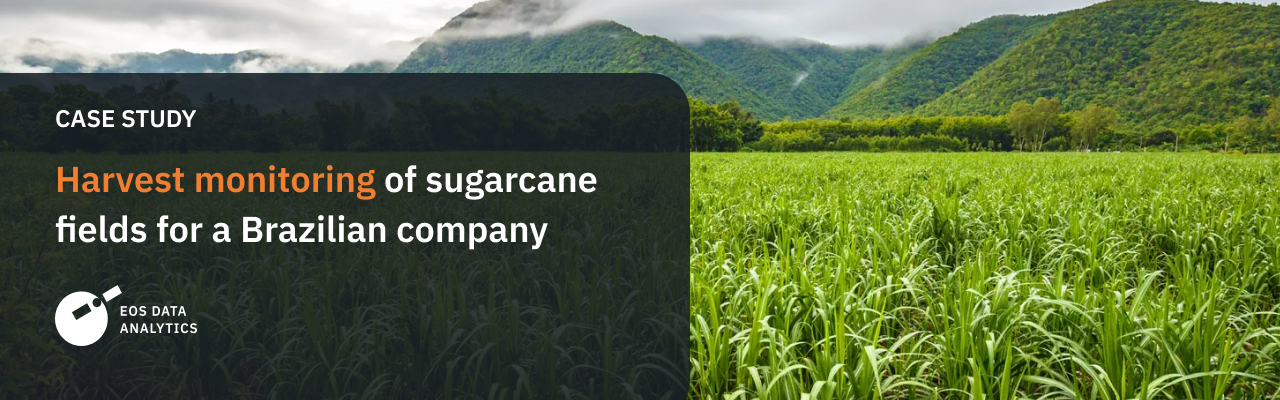
Sugarcane Harvest Monitoring In Brazil: Dates And Areas
Brazil is the top sugar exporter, supplying about 50% of all the world’s sugar. Agribusinesses and individual farmers make the produce from sugar cane. The crop is also used for producing ethanol — renewable fuel.
In this case study, we’ll find out how EOS Data Analytics carried out a custom project on sugarcane harvest monitoring for a Brazilian company.
Overview: Sugar Production In Brazil
The country’s agriculture businesses started transitioning from manual, outdated, expensive, and environmentally harmful pre-harvest burning (burning sugarcane leaves and tops, leaving sugar-bearing stalks only) to green cane harvesting. This alternative approach entails using machines with fans to clean leaves and tops from the stalks leaving the biomass on the ground to restore the soil. Thanks to this shift, farmers can streamline field operations and harvesting during the year, including the rainy season. The acceleration of sugar production activities is especially timely given the growing food demand and increased sugar consumption that comes with it. But novel methods of growing sugarcane and its harvesting require adopting technologies that would make it possible to evaluate production and continuously monitor farmlands for timely change detection.
Challenge: Labor-Intensity And High Cost Of Manual Field Inspections And Ground-Based Harvest Monitoring
When it comes to harvest monitoring on a regional or country level, the method that will overcome the coverage limitation of drone monitoring and drawbacks like the high costs of maintaining navigation equipment on farm machinery associated with ground-based harvest monitoring is needed. Using satellite data to monitor harvesting progress on large areas of sugarcane fields can be a rational solution. Determining harvest dates on a field level is one such project EOS Data Analytics worked on for a Brazilian customer.
Solution: Monitoring Harvest Dates And Calculating Harvested Areas Using Satellite, Meteorological, And Agronomic Data
EOSDA determined harvest dates and calculated harvested areas for a Brazilian company that needed this information for scheduling and optimizing logistic operations. The sugar manufacturer manages fields in the São Paulo state, which accounts for 60% of the country’s sugarcane production.
The team was working on the pilot project in 2019–2020 using records starting 2018 (it’s the year sugarcane was planted on monitored fields.)
Developing algorithms for monitoring sugarcane harvesting was justified by crop uniqueness and the difference in signs of harvesting compared with grain crops.
Sugarcane is planted twice a year, in winter (August–September) and summer (February–April) in very moist soil. The plant grows for one or one and a half years. In most cases, farmers harvest sugarcane in a green state and during dry periods in winter when saccharose concentration in plants is highest.
The specialists built two independent algorithms based on decision trees; Algorithm 1 was suited for analyzing time series multispectral optical data by Sentinel-2, while algorithm 2 was used to analyze time series satellite radar data from Sentinel-1 in combination with optical data.
The decision to use both types of satellite data was based on their properties and the partial availability of optical data.
Optical data is a good choice since sugarcane is harvested while green. Also, among the pros are the satellite’s frequent revisit time (five days) and high sensitivity of the reflection coefficients in spectral bands to vegetation changes within the fields. The disadvantage of optical imagery is that cloudiness and haze don’t allow for capturing the spectral characteristics of various channels used in the analysis.That’s when radar data comes in handy,” adds the scientist. “Moreover, radar satellite imagery gives us a larger window of opportunity because we can determine the texture of the field change. For example, after a crop is collected, a root area remains on a field (harvest residue), and its height and structure are no longer the same. The drawback of using radar data is its temporal resolution — 10–12 days.
Using optical data. To determine a harvest date using time-series optical data from the Sentinel-2 satellite, specialists analyzed images with NDVI as spectral reflectance of green vegetation, crop residue, and soil without vegetation differ. The index values increase gradually during plant growth and decrease sharply after harvest. And since sugarcane fields are harvested by parts (5–10 ha each), large fields were divided into square parcels to ensure the index decrease will be more detectable.
The best estimates of harvested areas per month were obtained for the period from March to August 2018, when a cloudy pixel percentage was less than 45% of the image area.
Using SAR data. Radar imagery can be used for clarifying harvest dates determined based on optical data and, as we mentioned, for specifying harvest dates when optical satellites’ cameras can’t capture the spectral characteristics of needed channels due to cloudy weather.
To monitor harvest according to the SAR data, we used coherence time series generated from Sentinel-1 IW SLC images. Low coherence corresponds to the period when harvest works take place. The coherence grows rapidly upon the harvest completion due to the bare soil or sparse vegetation.
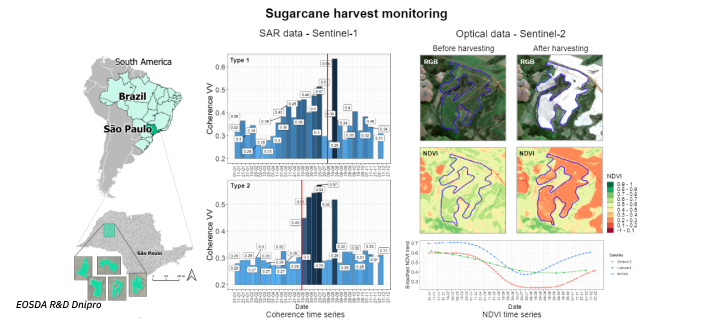
Besides satellite data, the team used agro calendar (sugarcane sowing dates) and weather data as using them together permits achieving a more accurate result.
To calculate the sizes of harvested fields, the team used a vector map containing polygons, each of a specific area, and defined changes within field borders using satellite imagery of these fields.
Using this technology, we can record partial harvesting of a field if necessary.
Outcome: Harvest Status, Dates, And Harvested Area Of 30 Fields Was Defined
The team prepared a digital vector map with 30 specified fields divided into 574 parcels, their harvest status, harvested area, and harvest dates. The total area of monitored fields was 3745 ha. The harvested territories identified by visual interpretation coincide with the optical-data algorithm (algorithm 1) by 97%; the coincidence with the algorithm based on radar and optical data (algorithm 2) is 90%.
Scientists achieved higher accuracy in defining harvest dates when working with optical data because the Sentinel-2 satellite’s revisit time was shorter (5 days) than that of Sentinel-1 (10–12 days). So, using data captured by both optical and radar satellites was justified.
After the pilot project ended in 2020, the client requested to monitor the progress of harvesting works in another province.
About the author:
Natalia Ivanchuk holds a Master’s degree in Applied Mathematics from the National University of Water and Environmental Engineering. She is an author of 60+ scientific publications, monographs, and other scientific works.
Natalia's expertise and constant desire to learn and perfect her programming skills (C++, C#, JS, Python), has been most beneficial for EOS Data Analytics.
Recent articles
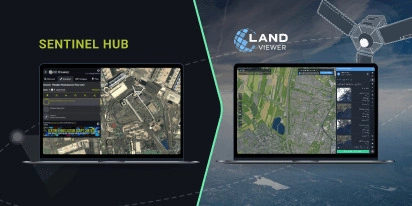
Transition Guide From Sentinel Hub EO Browser To EOSDA LandViewer
Need an EO Browser alternative that’s just as familiar but more flexible? EOSDA LandViewer offers free recent imagery, a vast satellite data archive, and advanced analytical tools.
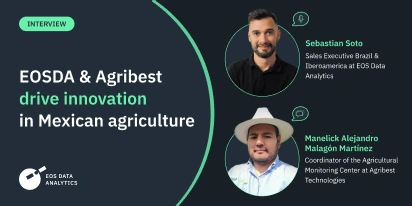
EOSDA & Agribest: Driving Agtech Growth In Mexico
In this insightful interview, Agribest shares how its collaboration with EOSDA is shaping Mexico’s agricultural future, with a focus on profitability, sustainability, and technology.
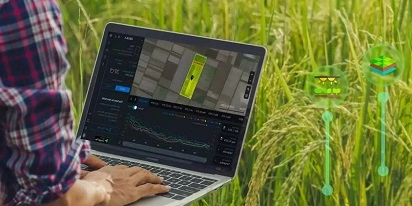
Top Ready-Made Agriculture Tools & Softwares for 2025
Agriculture softwares are silent partners in the field, working tirelessly behind the scenes to support farmers with insights and precision to cultivate sustainable agricultural ecosystems.