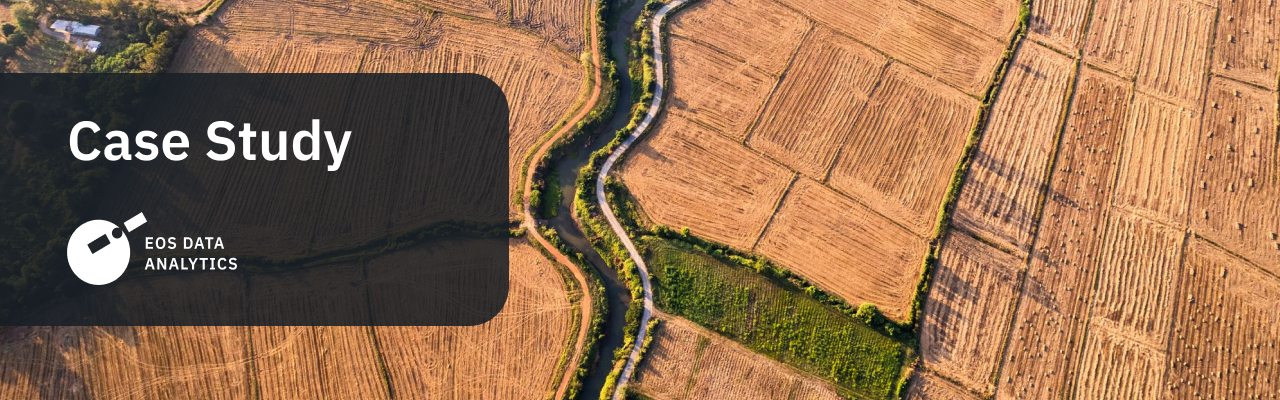
Crop Classification And Field Boundaries In Kyrgyzstan
Sugar production has been maintaining economic importance in a variety of countries across the world. While sugar cane clearly dominates the market, its crop competitor sugar beet shows good potential in terms of sustainable development.
The raw material of the latter accounts not only for ~20% of the world’s refined sugar amounts but also for other widely used products such as food for livestock and ethanol . In general, sugar beet production is currently connected with a multitude of industries like liquor, canned goods, confectionery, baking, pharmaceuticals, biofuels, and horticulture, to name a few. In fact, research shows that sugar beet growing practices lead to much fewer CO2 emissions than sugar cane while taking up less land and requiring less water .
A customer from Kyrgyzstan has reached out to the EOSDA team to obtain a custom-tailored crop classification map with field boundaries detection to be able to align their strategic production goals of sugar beet with real-life planning and resource estimation.
Overview: About Sugar Beet Production Dynamics In Kyrgyzstan
Sugar beet cultivation is one of the most important agricultural businesses in Kyrgyzstan. Multiple regions have dedicated large acreages of land in valleys to these crops and built sugar processing plants nearby. The amount of sugar produced has been showing stable growth while the local government plans to further modernize and support this industry in the coming years.
Optimizing production capabilities goes in line with global sustainable development goals and precision agriculture practices. For implementing new forward-thinking approaches, the EOSDA’s customer chose to start with detecting field boundaries and classifying crops with the help of space imagery and multi-level deep learning architecture.
Challenge: Analyzing Field Geoposition, Adjusting Logistics
A unique topography of sugar beet fields in Kyrgyzstan poses quite a few challenges for growers. Fields are situated mainly in the valleys that are surrounded by steep mountains. As a result, the area is well-moisturized. However, sugar beet is very demanding in terms of the condition of the arable horizon. Therefore, timely and high-quality soil cultivation is extremely important for the formation of a high yield of these root crops. A clearer understanding of field sizes and locations would help local sugar producers to adjust their cultivation technology throughout the seasons and plan the needed activities accordingly.
At the same time, business considerations are as important as agricultural precision per se. Research indicates that an average sugar extraction rate is 14% to 16% which means that only 140-160 kg of sugar is produced out of 1 ton of raw material . Therefore, it is not economically justifiable to design long and expensive logistical routes from fields to sugar plants. By viewing the field locations provided by satellite imagery, the client was able to plan the most efficient sugar production logistics.
Solution: Training A Neural Network Based On Unique Region Characteristics
Field boundary detection and crop type classification were chosen as deliverables for this project. The AOI (area of interest) was divided into a certain number of classes, according to crop types and/or geospatial characteristics (forest, water, bare land, etc.), and presented to the client in a vector format.
A custom neural network was supplied with ground truthing data, agricultural calendar, and satellite imagery data specific to the location. After that, it was trained on the basis of a supervised convolutional LSTM (Conv-LSTM) model, which allowed using the benefits of:
- Convolutional (CNN) neural networks: proven efficiency in image recognition and processing.
- Long short-term memory (LSTM) neural networks: able to work with long-term dependencies without the drawbacks of the vanishing gradient problem, which is especially handy for long agricultural cycles of vegetation.
Field boundaries can be described as polygonal shapes. EOSDA scientists supervised the model by providing information about expected field patterns, while the model itself was able to automatically define fields with an arbitrary number of points, thus identifying a variety of polygonal shapes that were also matched with particular crop types.
EOSDA Crop Monitoring
Using satellite monitoring for remote fields management in one platform!
The project implementation was carried out in late spring to the middle of summer 2021, at the highest vegetation stage of the season, which helped to obtain satellite imagery with clearly identifiable patterns.
The process of training a custom neural network model in EOSDA may vary depending on the individual inputs, project goals, and geographic location. In this case, the project was divided into a few major steps.
Step 1: Preprocessing
First, the EOSDA team obtained the input data, such as:
- AOI vector polygon layer
- Sentinel-2 data with relevant raster bands (10 meter resolution)
- Ground truth data in points (based on geolocation coordinates)
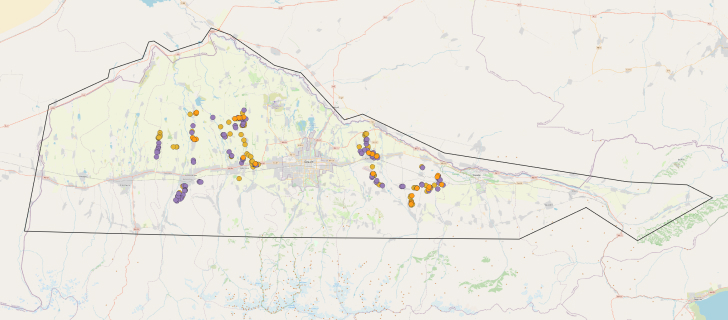
High-quality data is a necessary prerequisite for any machine learning algorithm. The EOSDA team obtained appropriate AOI satellite imagery data, including radiometric corrections of atmospheric noise and sensor irregularities, as well as geometric correction of distortions that result from variable positions of satellite sensors relative to the Earth. Potentially noisy data was filtered out before proceeding with the next step.
Step 2: Neural Network Model Training
The training process included two major stages:
- Classification model training on data provided by the client – locations of fields with certain crops. This model makes a classification: the output is a raster result where the image is clustered into classes according to crop cultures;
- Field boundary detection model adaptation. The output is a high-precision vector mask (the best possible result is usually picked individually or compiled out of the few best variants).
Once the EOSDA team obtained the crop classification and field boundary detection results, they were ready to assign the newfound values to a geographical map of the AOI.
Step 3: Image Interpolation
At this stage, the raster and the vector results from the two models are combined to get a better classification of crops in vector format.
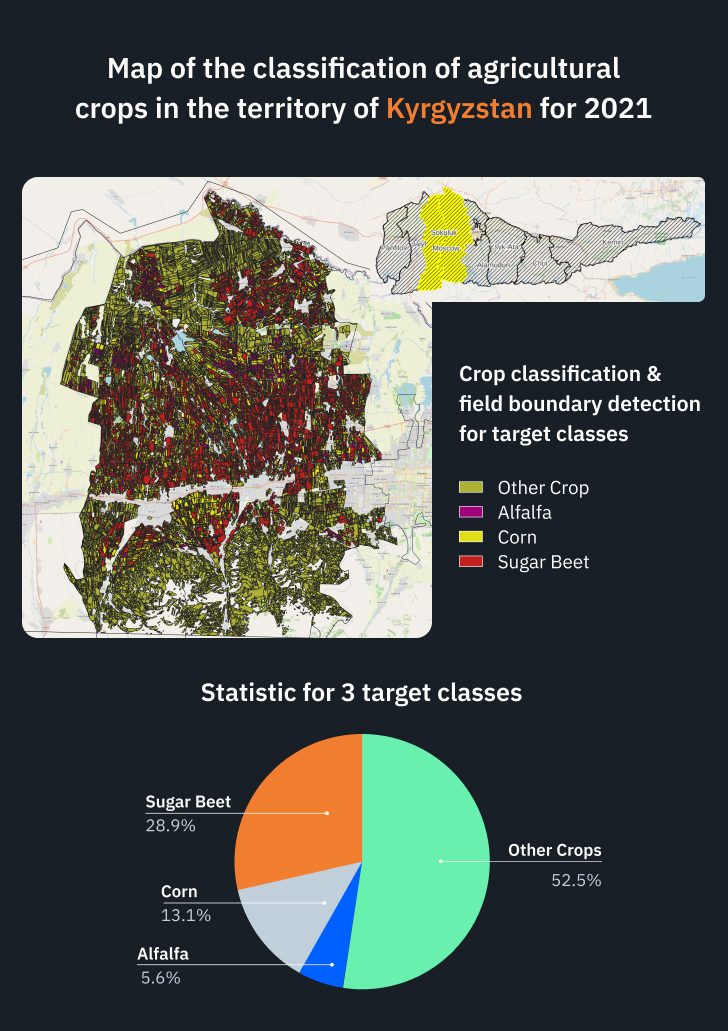
The output data demonstrated four crop classes that were previously negotiated with the client:
- Sugar beet
- Corn
- Alfalfa
- Other crops
Additionally, non-arable zones were detected, such as:
- Water
- Bareland
- Forest
- Grassland
- Garden
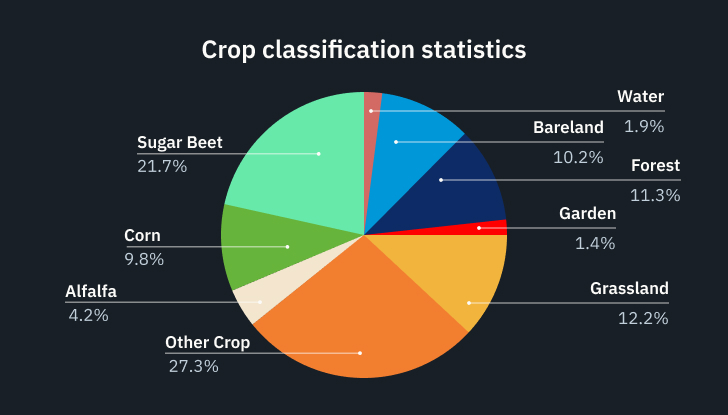
The automatically calculated acreage of each zone was provided in a spreadsheet format. The report also included statistical visualizations with result explanations and recommendations from EOSDA data scientists.
Outcome: Detailed Map Of Crops, Field Boundaries, Business Value
Crop classification and field boundary detection allowed the client to make realistic estimations about the projected amounts of sugar beet production in the region. Based on this information, they could:
- Remotely distinguish arable land from non-arable land;
- Identify the current location of sugar beet fields and plan the agricultural logistics accordingly;
- Calculate the actual acreage of particular crop types and assess potential yields;
- Conduct regional inventories based on precise geolocation;
- Estimate soil productivity in the given areas;
- Improve yield planning and assessment, while adjusting the strategies for meeting the import and export goals.
Newly acquired data has become the first step in embracing a wide array of possibilities for precision agriculture in Kyrgyzstan, thereby helping to realize the potential for sugar beet production development in the future.
About the author:
Natalia Ivanchuk holds a Master’s degree in Applied Mathematics from the National University of Water and Environmental Engineering. She is an author of 60+ scientific publications, monographs, and other scientific works.
Natalia's expertise and constant desire to learn and perfect her programming skills (C++, C#, JS, Python), has been most beneficial for EOS Data Analytics.
Recent articles
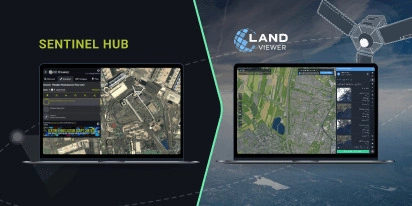
Transition Guide From Sentinel Hub EO Browser To EOSDA LandViewer
Need an EO Browser alternative that’s just as familiar but more flexible? EOSDA LandViewer offers free recent imagery, a vast satellite data archive, and advanced analytical tools.
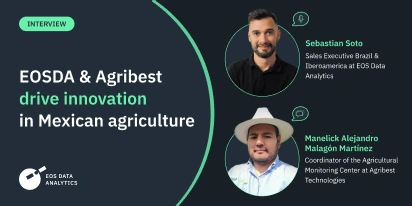
EOSDA & Agribest: Driving Agtech Growth In Mexico
In this insightful interview, Agribest shares how its collaboration with EOSDA is shaping Mexico’s agricultural future, with a focus on profitability, sustainability, and technology.
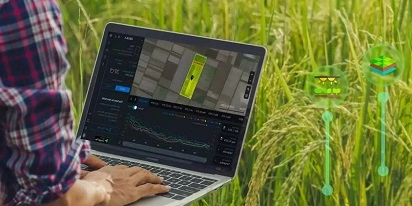
Top Ready-Made Agriculture Tools & Softwares for 2025
Agriculture softwares are silent partners in the field, working tirelessly behind the scenes to support farmers with insights and precision to cultivate sustainable agricultural ecosystems.