Crop Yield Prediction
EOSDA team of data scientists and engineers has developed effective techniques for crop yield estimation using remote sensing and machine learning models. We’re relying on earth observation data retrieved from satellites to cover areas ranging from individual farms to regions.
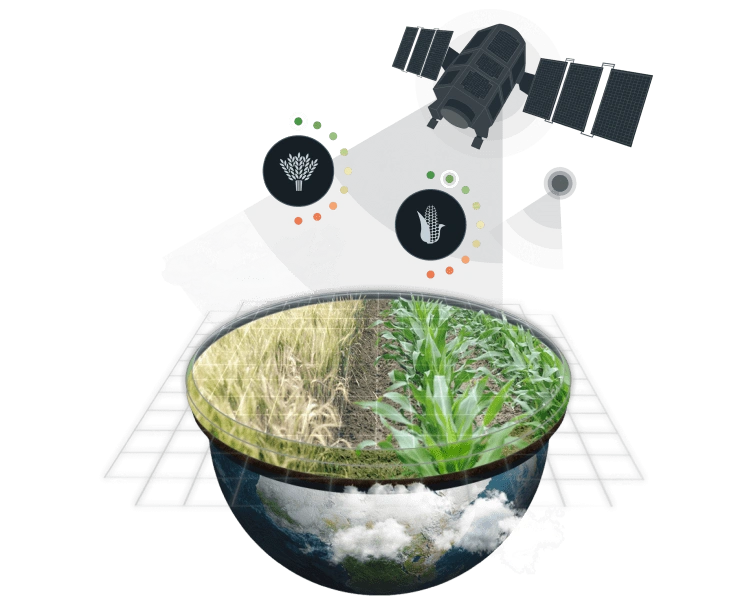
Crop Yield Prediction in Numbers
Accuracy
Forecasts
Crop types
Project speed
Entries per crop
Data Sources
Watch video about our yield prediction solution
Global food security depends on the efficiency of food management practices, such as yield prediction, which allows farmers to raise crops in a more sustainable way. EOSDA’s yield forecast solution – developed based on the latest technological advances in machine learning and geospatial analytics – provides farmers, agri holdings, food security companies and other decision-makers with crucial data needed for sustainable and profitable crop production.
Yield Estimation Benefits
- Increased speed of decision-making related to harvesting, storing, and transporting operations.
- Data on crop profitability in your area of interest based on yield estimation.
- Opportunity to strengthen global food security by introducing crop yield forecasting to developing countries - helping them to prevent famine, boost local economies, and implement sustainable agricultural practices.
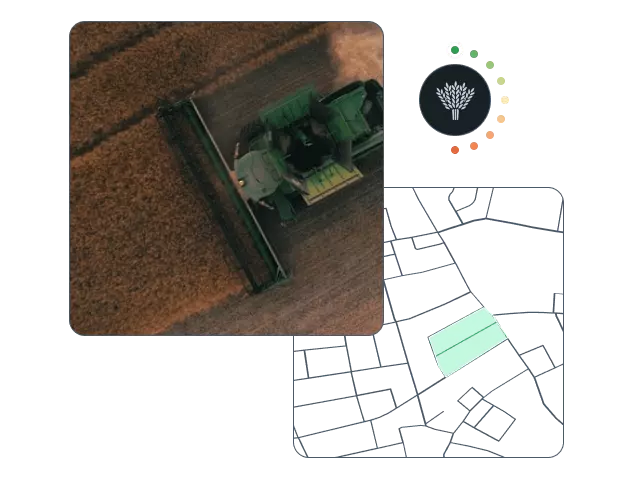
- Improved understanding of the agricultural market and better-informed decisions on the management of stocks, imports, and exports, in accordance with CAP and other similar policies.
- A much better understanding of cumulative effects of hostile field conditions (pests, diseases, nutrient deficiencies, and others) on crop development.
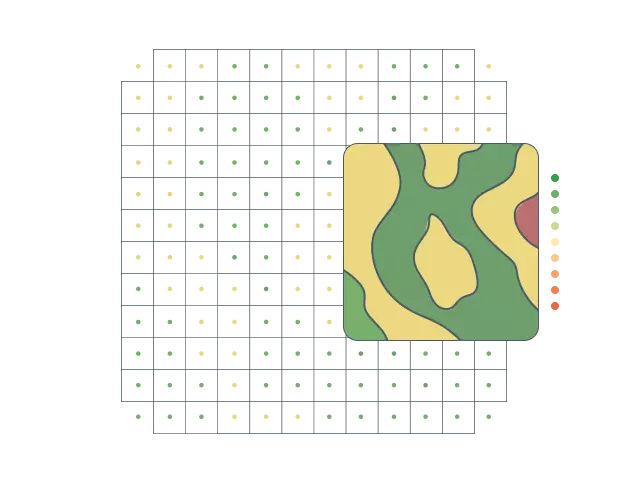
Our Approach
Biophysical yield prediction model
- Collect data (weather parameters, soil analysis, crop state, phenological data, etc.).
- Calibrate the model and carry out the LAI assimilation to ensure accuracy of a crop yield forecast in the absence of statistical data and to increase the variability of values.
- Simulate the biological productivity parameters (TAGP, WSO, relative soil moisture, total water consumption, and others) to estimate yield.
- Update the data once every 14 days to increase the accuracy. This has to do with weather updates.
Statistical yield prediction model
- Collecting data to create a crop yield prediction dataset and combining it with possible predictors (rainfall, temperature, humidity, soil type, and others).
- Picking the right ML model for the project - e.g. Linear regression, Random Forest, LightGBM, XGBoost, CatBoost, to name a few.
- Adjusting the model to answer the specific needs of the project in question for best results.
-
Ensemble of model scenarios
-
Asquisition 1
-
Asquisition 2
-
Asquisition 3Selection of most likely scenario and re-initalization of the modelled ststem state with the scenario
-
Asquisition 4Observations of LAI
-
Asquisition 5
-
Harvest
Applying LAI assimilation allowed us to achieve the 95% accuracy in 30% of the fields. For the fields marked in red, the accuracy of less than 80% was achieved, while the crop yield forecast accuracy for the green-marked fields exceeded the 80% mark.
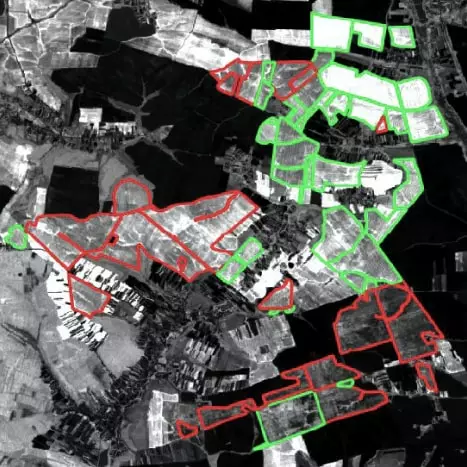
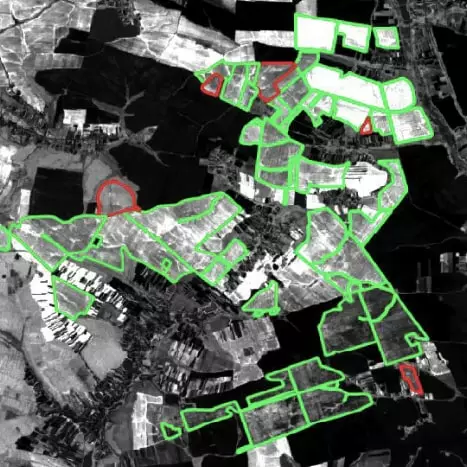
Our Success Stories
Yield forecast for a large agroholding in Ukraine
- 45 days prior to the harvest
- 2 weeks prior to the harvest.
- less than 80% accuracy
- more than 80% accuracy
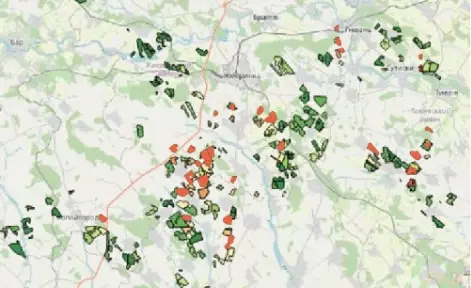
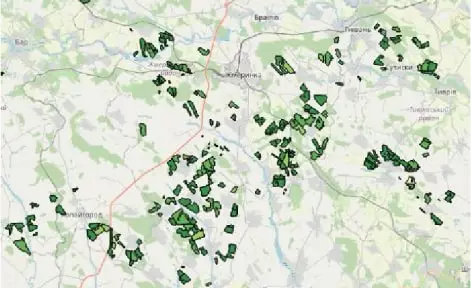
Accuracy (Wofost) | Accuracy (Wofost + Lai) | |
---|---|---|
Maize | 0.75 | 0.91 |
Soy | 0.78 | 0.86 |
Sunflower | 0.71 | 0.88 |
Winter barley | 0.53 | 0.82 |
Winter wheat | 0.75 | 0.92 |
Number of Fields | ||||||
---|---|---|---|---|---|---|
Crop / Accuracy | <70% | 70-75% | 75-80% | 80-85% | 85-90% | >90% |
Winter barley | 27 | 7 | 5 | 22 | 23 | 52 |
Winter wheat | 33 | 17 | 19 | 21 | 19 | 102 |
Winter rapeseed | 26 | 6 | 20 | 14 | 27 | 22 |
Sunflower | 12 | 11 | 12 | 14 | 19 | 22 |
Soy | 28 | 22 | 29 | 58 | 37 | 86 |
Crop yield forecasting for a Canadian insurance company
Goal: Reliable predicted yield data on every customer to reduce insurance risks.
Input data: Over 100 fields on 20 farms.
-
Canola> 98,03
-
Corn> 87, 59
-
Peas> 76,25
-
Soybean> 95,94
-
Sunflower> 98,21
-
Wheat> 98,63
Crop | Modelled Yield | Actual Yield |
---|---|---|
Canola, lbm/ac | 41,81 | 41,00 |
Corn, BPA | 123,65 | 110,00 |
Peas, q/ac | 30,94 | 25,00 |
Soybean, BPA | 22,89 | 22,00 |
Sunflower, lbm/ac | 1767,73 | 1800,00 |
Wheat, BPA | 53,72 | 53,00 |
Grand Total | 95,6 | 94,47 |
-
Canola> 96,96
-
Corn> 91,69
-
Oats> 99,98
-
Rye Fall> 85,85
-
Sunflowers Confects> 85,36
-
Sunflower Oils> 98,06
-
Wheat> 94,95
Crop | Modelled Yield | Actual Yield (Farm 4) |
---|---|---|
Canola | 40,19 | 39,00 |
Corn | 119,14 | 110,00 |
Oats | 125,03 | 125,00 |
Rye Fall | 64,39 | 75,00 |
Sunflowers Confects | 2063,60 | 1800,00 |
Sunflower Oils | 1834,19 | 1800,00 |
Wheat | 61,73 | 65,00 |
Grand Total | 584,34 | 528,00 |
Field name | Canola | Corn | Soybean | Sunflower Oils | Wheat |
---|---|---|---|---|---|
SE-2-6-28-W1 | 58,68 | 194,33 | 41,45 | 2208,85 | 72,49 |
SW-36-7-28-W1 | 30,91 | 169,49 | 14,42 | 1146,91 | 46,24 |
W-34-5-27-W1 | 38,77 | 151,58 | 24,71 | 1476,83 | 59,82 |
The harvest period for the target crops in Canada usually lasts from August till September. Knowing this, we were able to forecast yield two months before the harvest, achieving an accuracy of over 82%. The accuracy was steadily increasing as the harvest was approaching until it reached 90% just two weeks prior to the harvest, as had been expected.