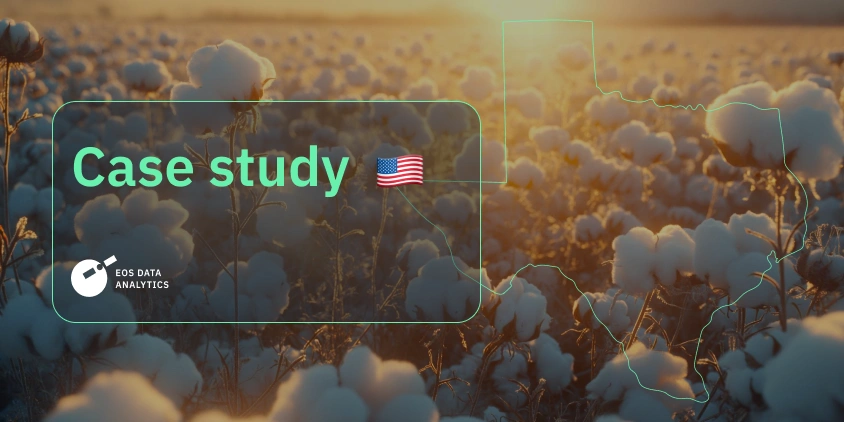
Cotton Yield Estimation In Texas: Proof Of Concept
For centuries, farmers wanted to know the possible yield of their fields in order to choose the most profitable crop and minimize the risks of various negative factors. Now, precise yield estimation and prediction can be done in various ways, one of which is using artificial intelligence and machine learning algorithms. EOS Data Analytics (EOSDA), a global provider of AI-powered satellite imagery analytics, developed a custom solution for crop yield prediction. This tool allows the company to predict crop volume accurately using remote sensing and machine learning (ML).
EOSDA has recently completed a custom project to ensure the model applies to cotton. The Yield Prediction model needed to be adjusted according to the specifics of this crop, so the team gathered datasets, rolled up their sleeves, and got down to business. Here’s how it went.
Challenge | Predicting cotton yield in Texas was no easy task, as the model lacked data. The EOSDA team used the datasets they had for five counties. |
Solution | The team chose the Random Forest Regression model, gathered all data they could find, and adjusted the Yield Prediction model so that it would accurately predict cotton yields. |
Outcome | They managed to predict the yields with 80% accuracy. The model has the development potential. |
Overview: Texas Cotton Production
Cotton is used everywhere, from a T-shirt to a dollar bill. It accounts for acres upon acres of arable land, and the United States is the third leading cotton-producing country and one of the largest exporters in the world . In the US, Texas is responsible for 40% of the cotton production . In the state, many generations of farmers have grown this crop for centuries.
However, the situation changes. Heat waves, droughts, and other natural phenomena create harsh conditions for growing cotton, causing farmers and agribusinesses to lose billions of dollars . We’re talking not only about the direct impact on crop yields but also about planting schedule disruption, water resource depletion, and increased prevalence of pests and diseases.
Because of such intense climate change, farmers are forced to adopt new agricultural practices, invest in costly irrigation systems, and obtain tools that can accurately predict droughts or show their effects so they can act as early as possible. One tool that can help cotton producers battle climate change consequences is estimating and predicting yields.
Yield prediction data can really help in many ways. It can ensure resources are used efficiently, improve financial planning, and keep the supply chain running smoothly. Accurate forecasts can help reduce risks, guide market strategies, and meet contracts. Overall, this leads to smoother operations, better financial health, and a stronger position in the market.
EOS Data Analytics’ team of scientists decided to check their yield prediction model on cotton to ensure its accuracy.
Challenge: Not Enough Data For Cotton Yield Prediction
The science team needed to test the Yield Prediction model to ensure it worked for cotton with satisfactory accuracy across different counties, given the differences in crop growth conditions and the availability of data. Since the model required many different datasets, the team combined historical weather data, crop calendar information, crop yield statistics, and other relevant information.
There wasn’t enough data itself for Texas. There was information on four or five years of prior crop data for several counties, but it was not precise enough to apply our current model. The project would go much smoother if the dataset was based on fields with the known sowing dates.
With the data at hand, however, the objectives of the model testing on cotton were as follows:
- Check the accuracy of the model;
- Find potential challenges and ways to improve the model for the area of interest (AOI);
- Make an informed decision about further expansion of the project.
Solution: Model Adjustment
The research focused on estimating the crop yield for 2023. The area of interest, as per the available data from the USDA’s National Agricultural Statistics Service, Texas Field Office (Part of the Southern Plains Regional Field Office), was five central cotton-producing counties — Lubbock, Hale, Lynn, Crosby, and Nueces. These areas were chosen for their significant cotton production and the availability of high-quality satellite imagery. Their borders can be seen on the maps below.
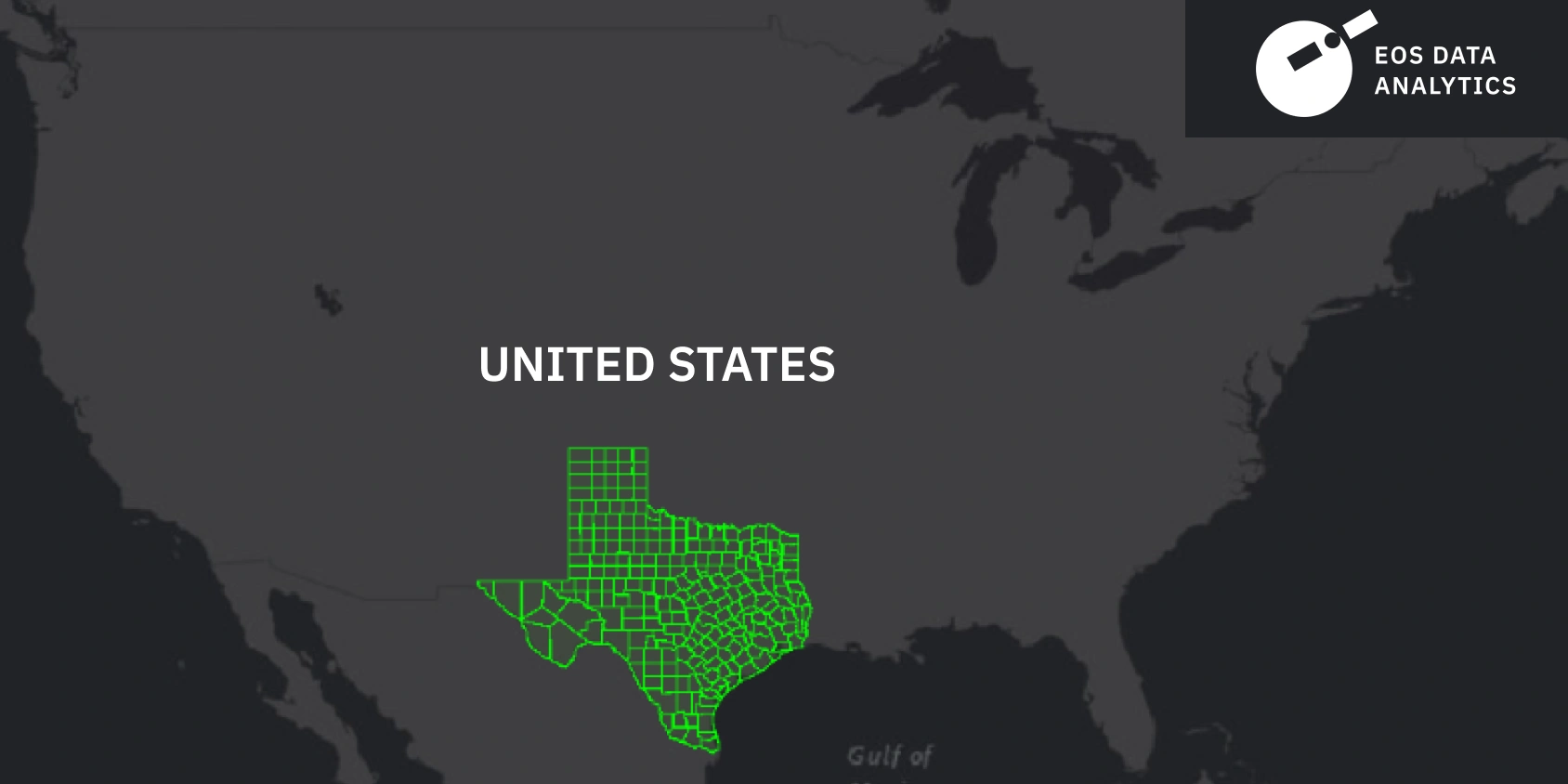
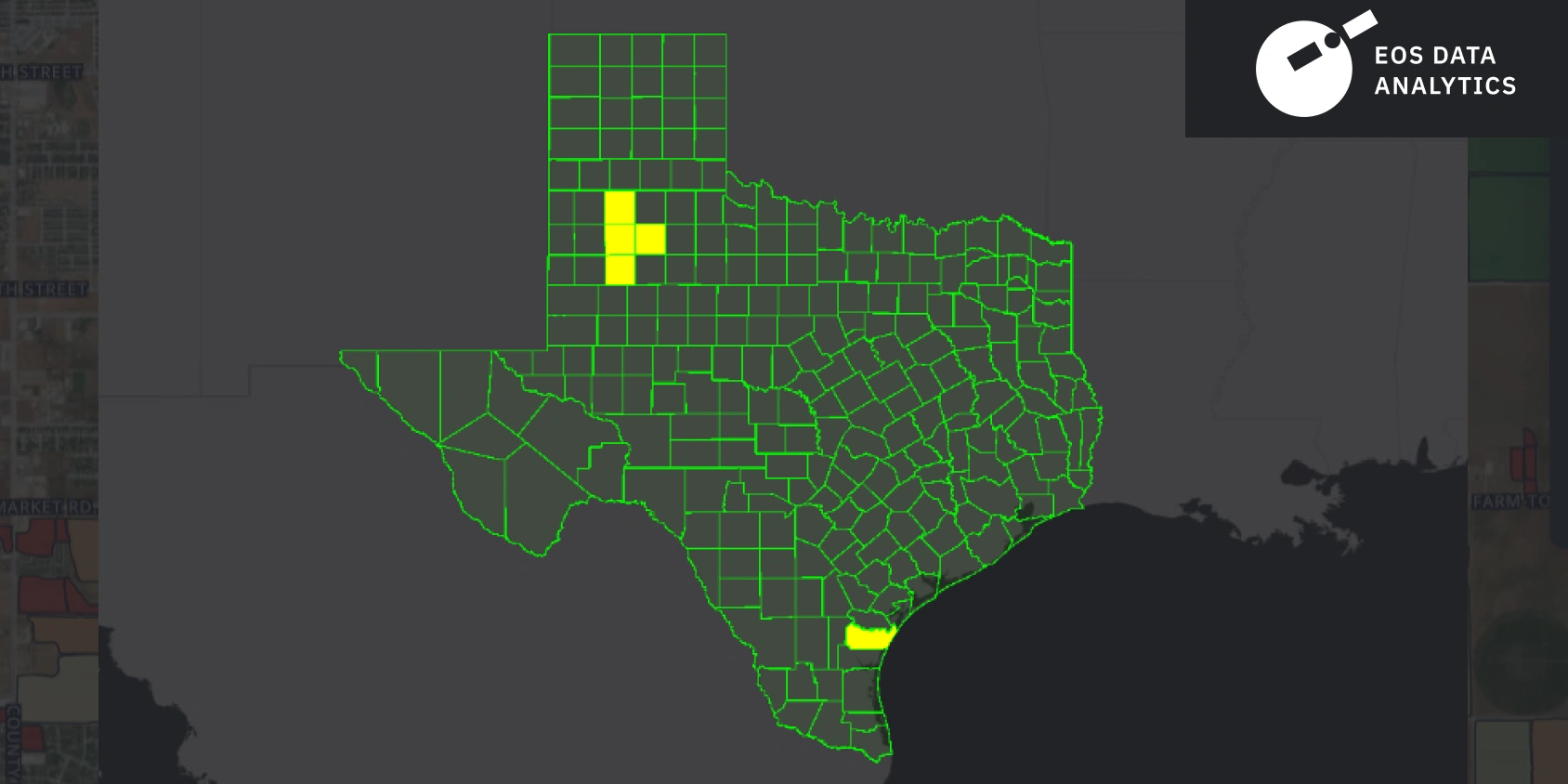
The data used in the project included crop yield statistics, weather data, crop calendar data, and other input datasets, like raster result and field boundary layer.
Crop yield statistics, as mentioned, were taken from the USDA’s National Agricultural Statistics Service for the target counties from 2020 to 2022.
Weather information, however, required data for the same period within the cotton crop season from the Copernicus Climate Data Store platform. This data included average monthly data on temperature at the level of 2 m, evaporation, solar radiation, soil surface temperature at two levels of 0–7 cm and 7–28 cm, solar radiation, precipitation totals, and soil moisture at four levels of 0–7 cm, 7–28 cm, 28–100 cm, and 100–289 cm.
Crop calendar data was publicly available on the cotton crop calendar in Texas and its counties. The growing period from March to October for each season from 2020 to 2023 was analyzed. Other input datasets were used as well, like cotton production at the field level. Those datasets included raster result of crop classification and vector layer with detected field boundaries for the entire AOI, both performed by the EOSDA team earlier.
To estimate cotton yield for target counties in this project, the science team, along with the data engineering and data analysis teams, chose the Random Forest Regression model. This model was selected due to its efficient calculations and ability to work with limited aggregated input data. Random Forest Regression is a supervised learning algorithm and bagging technique that uses ensemble learning for regression in ML — the trees in a random forest run in parallel without interacting during their construction. The main predictors in the model were weather data for the growing seasons aggregated on a monthly level and soil and phenology data for the period of 2020–2023. The target variable was statistical yield data at the county level for the same years.
Simple mathematical operations were employed for production calculations in this project. Specifically, the yield value obtained for each county was multiplied by the number of acres of each detected field. The number of acres was determined from the vector mask with the field boundaries. Thus, the production of a particular field in a specific county was calculated by multiplying its area in acres by the yield in pounds per acre for that county.
The project was carried out in several main stages, each with essential details. The EOS Data Analytics team began by analyzing the characteristics of the climatic zone, vegetation, and terrain in the area. This was followed by gathering necessary input data, including crop yield statistics and weather data. The model was then trained using data from the 2020–2022 seasons. The final step was the yield modeling to estimate cotton yield for each target county in 2023.
The production calculation step included several post-processing tasks. The team filtered the crop classification results by target counties, excluding all non-target crops and retaining only cotton. These results were then vectorized and combined with field boundary detection data. Next, they calculated the area of each detected cotton field in the target counties and performed production calculations for each field according to the previously described methodology.
The final step was to prepare the final vector layers, create visualization maps, and compile the project report.
The model itself is not unique, but the number and quality of predictors differ from one company to another. The data used to build the connection of the model with our target predicted yield value is different in each case.
Outcome: Accuracy In Cotton Yield Prediction
The project report showed the visualization of the results, as can be seen in the images below.
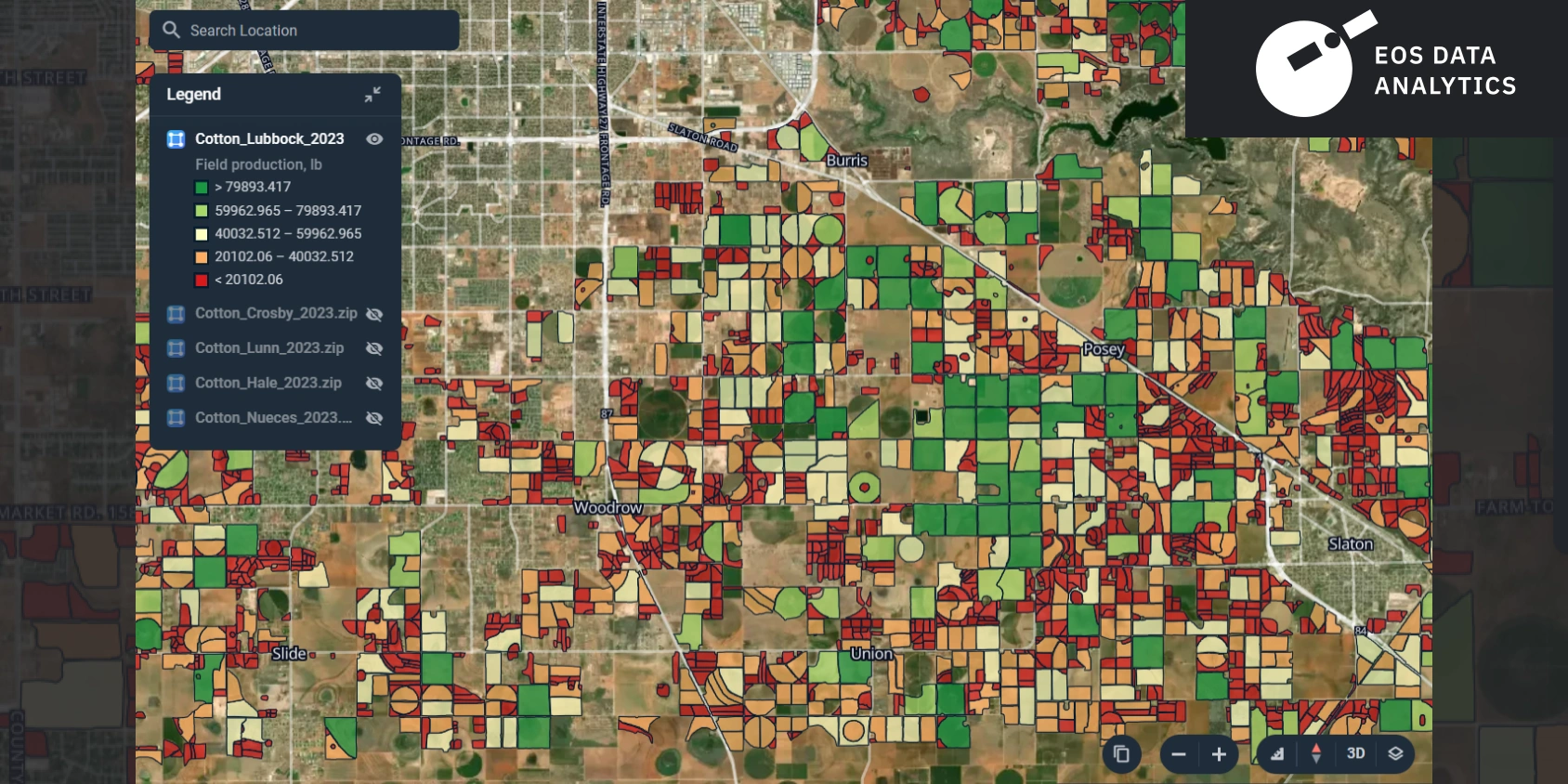
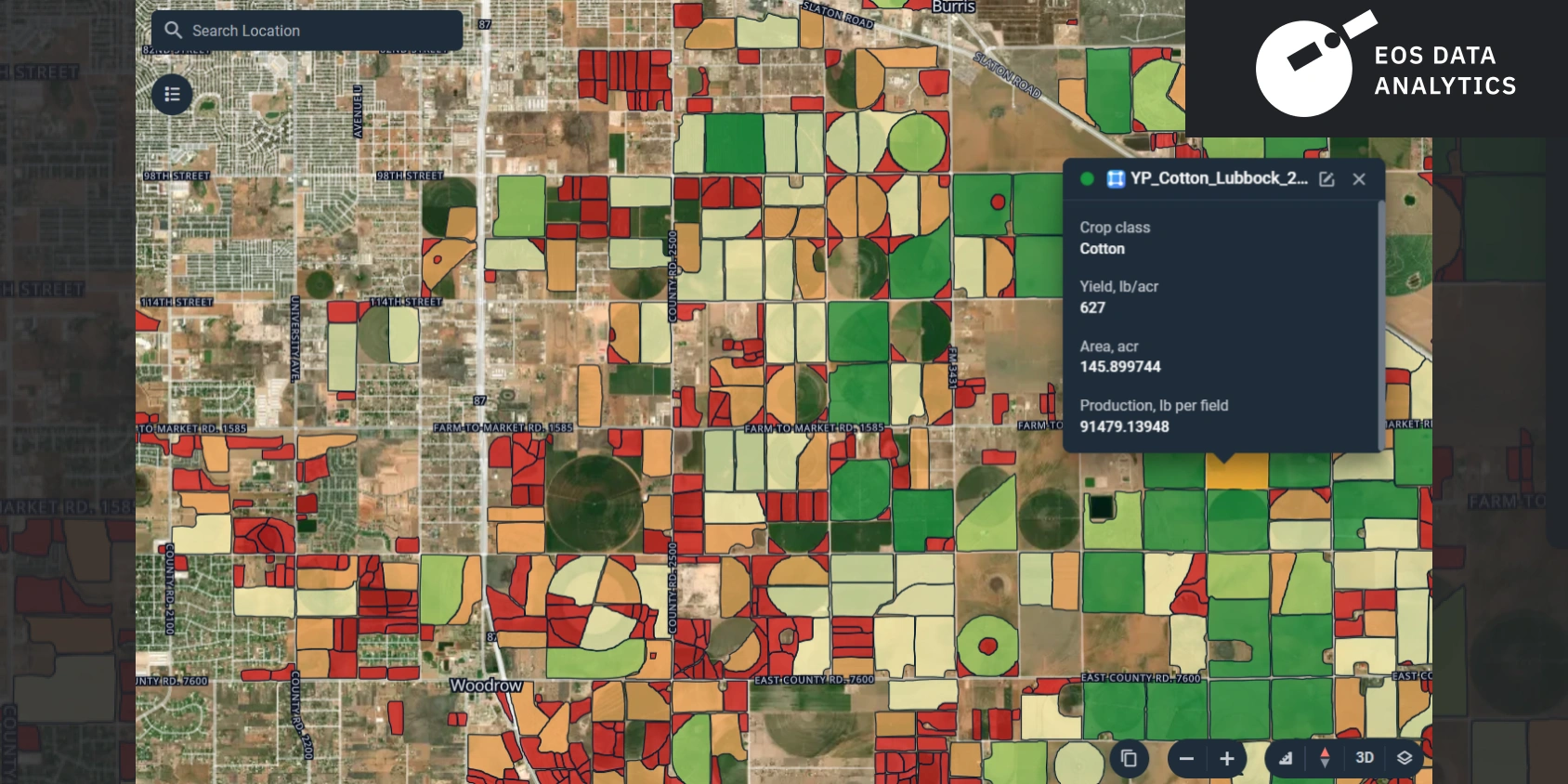
The accuracy of the test sample was about 80%, and it was evaluated using the cross-validation approach. This level of accuracy can be considered satisfactory for yield estimation and prediction models. The project proved that EOS Data Analytics automates ML and AI processes, saving time and resources usually spent on manual data collection and analysis. This helps our partners stay competitive in the commodities market.
There are certain perspectives for scaling the model as well. The approach used can be scaled up to larger areas if necessary or shrunken down with the appropriate amount of data. The more predictors the model uses, the more accurate it becomes. For instance, adding temperature, relative soil moisture, total or ground production, total water requirements, and consumption would only enhance the yield prediction model precision.
While biophysical modeling and satellite data assimilation, like WOFOST, were not used in this project, incorporating such models could be a future step to enhance accuracy and serve as an additional predictor for yield estimation.
We are a satellite-based data company, so in this model, we can also use average vegetation indices, like normalized difference vegetation index, leaf area index, or normalized difference water index. Those indices can only be accessed from above, from satellite imagery analytics.
Cotton yield estimation is possible in Texas, as this case study proves. Farmers and agribusinesses can easily predict their crop levels using services like Crop Yield Prediction. Prospects for developing this model are vast, so our team cannot wait to test them all.
About the author:
Kseniia Kunakh has over 6 years of writing experience, working in various domains, including business, educational, and media-directed texts. Kseniia’s previous experiences as a development manager in a Ukrainian eco-NGO and as a talent matcher in an IT company make her a perfect combination of someone who is passionate about eco-tech innovations and can communicate about them with ease.
Recent articles
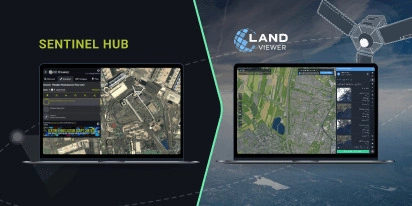
Transition Guide From Sentinel Hub EO Browser To EOSDA LandViewer
Need an EO Browser alternative that’s just as familiar but more flexible? EOSDA LandViewer offers free recent imagery, a vast satellite data archive, and advanced analytical tools.
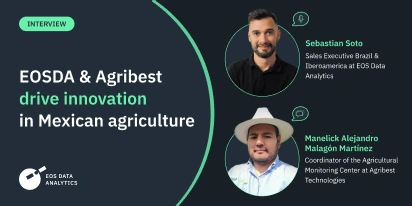
EOSDA & Agribest: Driving Agtech Growth In Mexico
In this insightful interview, Agribest shares how its collaboration with EOSDA is shaping Mexico’s agricultural future, with a focus on profitability, sustainability, and technology.
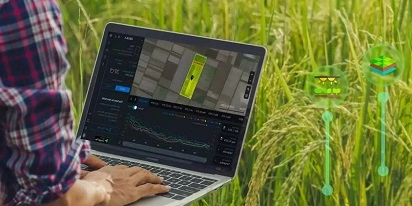
Top Ready-Made Agriculture Tools & Softwares for 2025
Agriculture softwares are silent partners in the field, working tirelessly behind the scenes to support farmers with insights and precision to cultivate sustainable agricultural ecosystems.