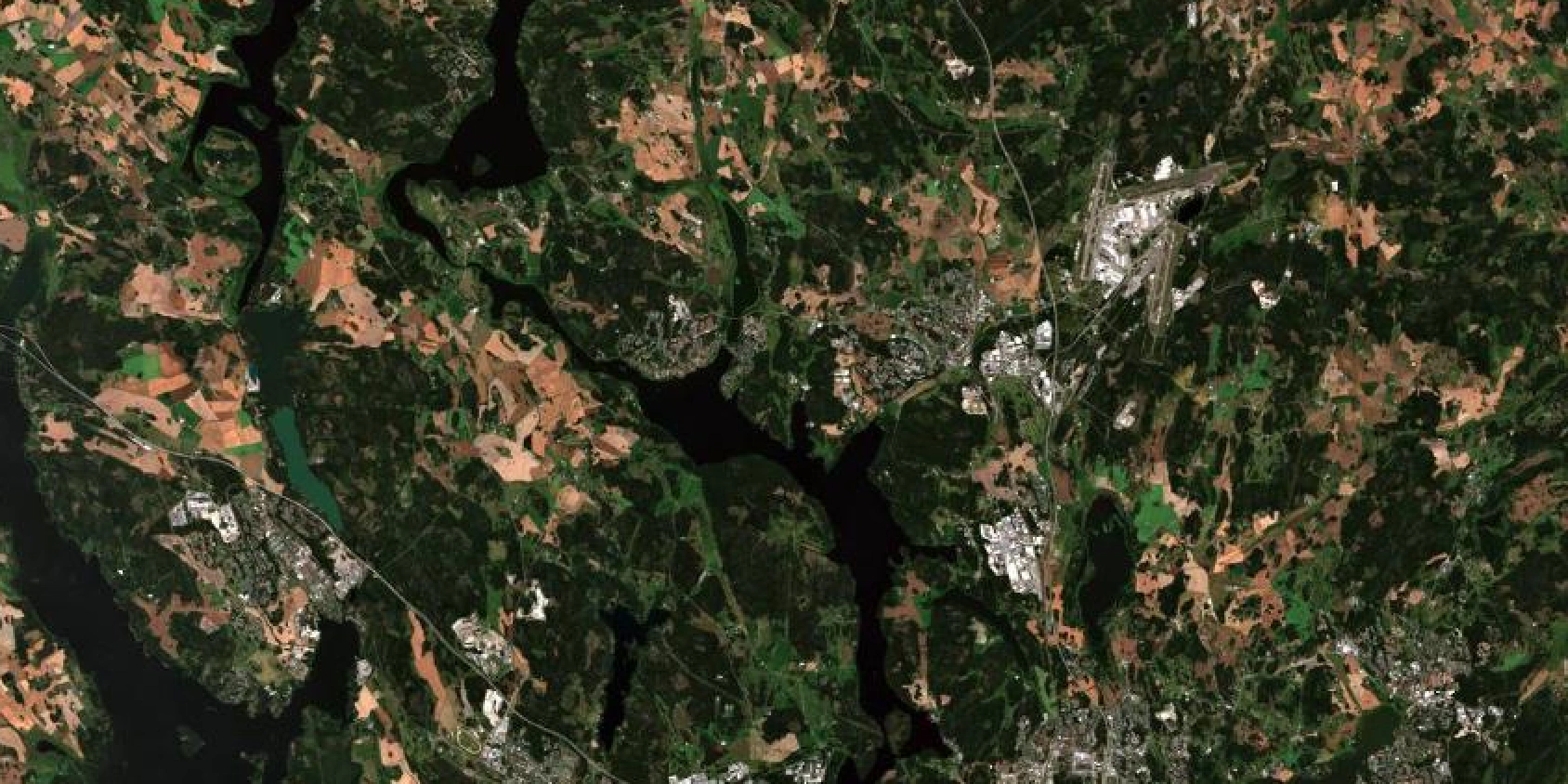
How To Interpret Satellite Images: Methods And Elements
Satellite image interpretation unlocks crucial insights for commercial users in the agriculture, forestry, and sustainability sectors. By properly reading space-derived data, they can make informed decisions about their land and resources. How to interpret a satellite image? Consider the elements visible in the image, such as shape, texture, and pattern, and what they convey both individually and in combination.
Online platforms now streamline the satellite image interpreting process, making powerful tools accessible to wider audiences. With the right instruments, even novices can translate live raw satellite imagery into practical information for guiding their land management and sustainability practices.
What Is The Process Of Satellite Image Interpretation?
Satellite image interpretation is a sophisticated process that involves extracting meaningful information (both quantitative and qualitative) from space-derived imagery. In a nutshell, when you interpret images, you transform raw visual data into valuable insights about objects and phenomena on Earth, which aids decision-making in many spheres.
The process of interpreting real-time and historical satellite imagery relies on several key techniques:
- Detection and identification entail recognizing specific targets within an image. Analysts identify various features such as vegetation types, soil compositions, or water bodies. The level of extracted detail directly correlates to the spatial and spectral resolution, with higher resolutions enabling more precise identification.
- Delineation, the stage of manual satellite imagery interpretation that occurs once targets are identified, implies outlining them for mapping purposes. This interpreting technique is essential for creating accurate maps of land cover, urban areas, or natural resources. Identification and delineation are the foundation of image classification, a process that divides complete images into discrete categories.
- Enumeration focuses on counting specific phenomena within an image. Building upon detection and identification, enumeration allows analysts to quantify features such as the number of trees, buildings, or vehicles in a given area. This step in interpreting imagery is particularly valuable for forestry management, urban planning, and traffic analysis.
- Mensuration, perhaps the most comprehensive technique of satellite imagery interpretation, involves measuring various aspects of identified targets. This can include area calculations, volume estimations, and linear measurements. Commercial applications range from assessing crop yields and timber volumes to measuring urban sprawl and infrastructure development.
Next, we’ll examine the key elements that guide analysts in applying these techniques for interpreting images effectively.
What Are The Elements Of Satellite Image Interpretation?
Visual interpretation of satellite images is a fundamental skill that allows analysts to extract valuable information from Earth observation data. By studying specific characteristics of objects and features within these images, users can gain profound insights into the Earth’s surface and its changes over time. These are the main elements of satellite images and their interpretation to focus on:
- Shape refers to the external form or outline of an object. For commercial users such as large-scale farmers or forest managers, interpreting shapes can help identify different land use patterns, track changes in field boundaries, or monitor forest encroachment.
- Size perception depends on image scale and resolution. Higher resolution lets viewers identify smaller features, needed for the precise asset mapping or monitoring changes over time.
- Pattern, simply put, is the repeated spatial arrangement of objects. Sustainability-focused businesses may use the visual interpretation of satellite image patterns to identify agricultural and urban sprawl or analyze transportation networks for efficiency improvements.
- Shadow can reveal the height and structure of objects. For instance, forestry professionals can interpret shadows to estimate tree height.
- Tone, the relative brightness or color of objects, is particularly useful for identifying different types of land cover and assessing vegetation health.
- Texture represents the frequency of tone shift, visualizing surface roughness/smoothness. Landowners can use texture analysis to assess soil conditions or identify eroded areas.
- Location (association) is the spatial context of features relative to their surroundings . For commercial users, interpreting location relationships can inform site selection for new projects or help optimize resource allocation in existing operations.
- Resolution is the level of detail and precision discernible when reading a satellite image. High spatial resolution is particularly valuable for precision agriculture, while high spectral resolution allows for a more detailed analysis of surface properties and conditions.
Understanding these elements is crucial for accurate interpreting and informed decision-making across industries and applications.
How To Interpret Different Types Of Satellite Imagery In EOSDA LandViewer
Modern satellites collect data across multiple spectral bands, providing a wealth of information about Earth’s surface beyond what’s visible to the naked eye. Interpreting these different band combinations unlocks new perspectives on everything from crop health to urban sprawl. EOSDA LandViewer brings this sophisticated technology to your browser, offering an intuitive platform for accessing and interpreting satellite data. With its extensive data library and built-in analysis tools, our platform streamlines the process of finding, visualizing, and understanding different types of satellite data for various applications.
True Color Satellite Images
Images of true color, also known as natural color, offer a view of Earth as we’d see it with our own eyes. What do natural color satellite images show? By using visible light spectrum data, based on red, green, and blue (RGB) colors, this band combination creates imagery where features appear in familiar colors. Interpreting such data is intuitive even for novices: healthy vegetation shows up as green, while stressed plants appear brown or yellow. Recently cleared fields stand out as very light areas, and man-made structures like roads are easily identifiable in gray.
One of the strengths of true color imagery is its ability to penetrate water, providing information about sediment and bathymetry. It’s also easy to interpret for the purpose of studying natural landscapes and urban areas. Researchers and urban planners frequently interpret these images to track city growth and changes in built-up regions over time.
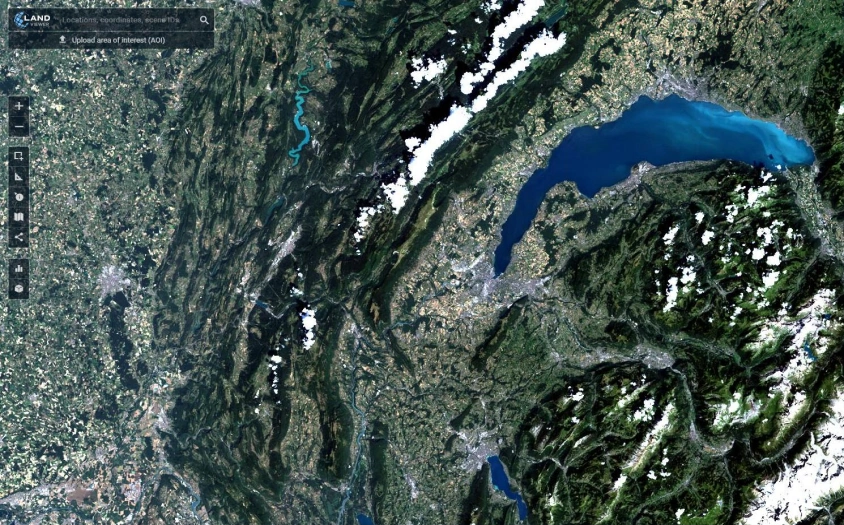
Color Infrared Satellite Images
Color infrared, or false color, imagery detects the varying levels of infrared radiation reflected by different surfaces, revealing previously invisible details about our environment. False color images incorporate infrared data by assigning visible colors to infrared wavelengths . This helps highlight certain features, such as vegetation, which reflects near-infrared light strongly and appears red in the false color.
How to interpret color infrared satellite images? To properly interpret them, you should know how infrared and visible colors correspond there. The table below covers the typical color assignments used in color infrared imagery to distinguish different environmental and urban features.
Feature | Appearance in false color |
---|---|
Healthy vegetation | Deep red |
Grasslands and sparse vegetation | Light red |
Coniferous and broadleaf forests | Deep red |
Soils | Dark to light brown |
Urban landscapes | Cyan blue |
Clear water | Black |
Snow, ice, and clouds | White or light cyan |
By interpreting color patterns of infrared satellite images, observers can gain comprehensive insights into soil and vegetation health, optimize water management, and monitor environmental changes. That’s why it is crucial to correctly interpret and analyze false color infrared imagery in sustainable land use and conservation efforts.
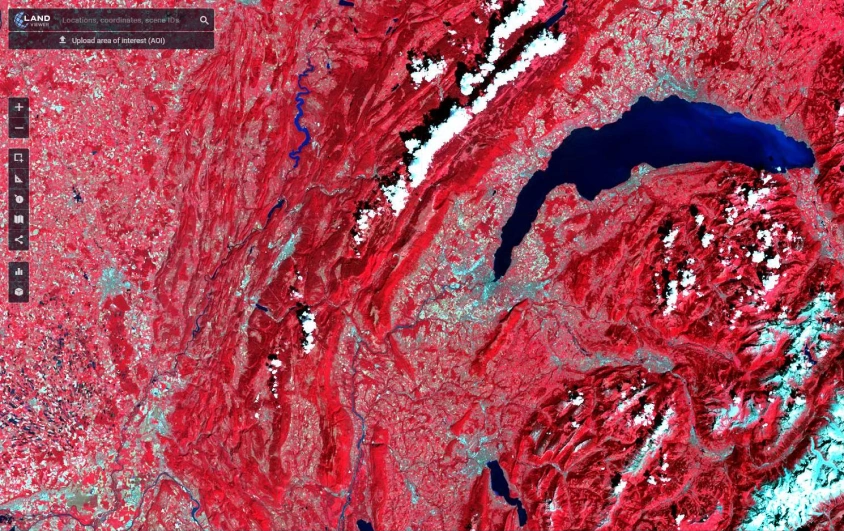
NDVI-Based Satellite Images
Imagery based on the Normalized Difference Vegetation Index (NDVI) uses plants’ unique spectral properties to show users the health of their crops or forests at a glance, across thousands of acres. This technology calculates a vegetation index by mathematically comparing near-infrared and red light reflectance to reveal plant health and density. The resulting NDVI values range from -1 to 1, with higher values indicating more robust vegetation.
For commercial users, interpretation of NDVI-based satellite imagery is invaluable. Food producers and agricultural cooperatives can optimize crop management by identifying areas of plant stress, predicting yields, and planning harvests. Forest managers can assess overall health, detect pest infestations, and monitor recovery after disturbances. Carbon project developers can interpret NDVI imagery to track reforestation projects and quantify carbon sequestration efforts.
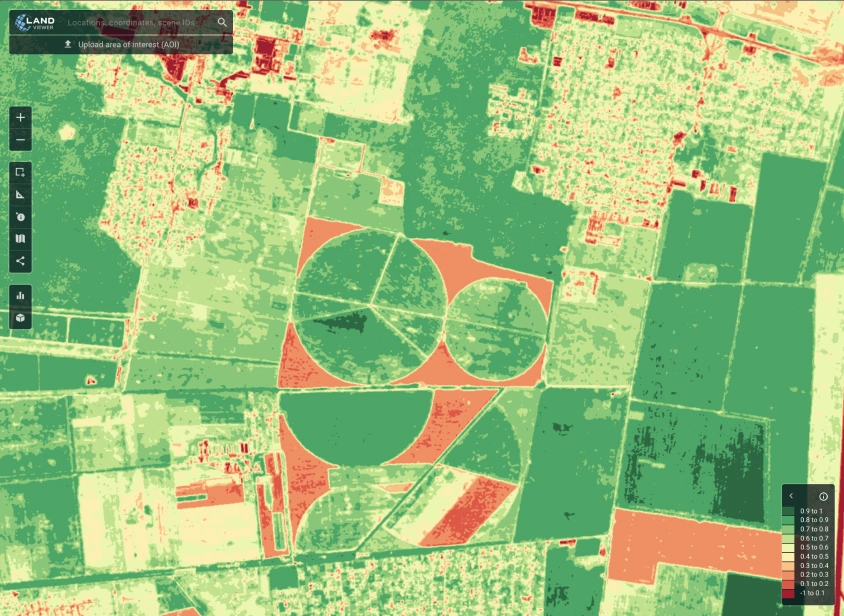
Forestry (Deforestation) Index Satellite Images
The forestry (deforestation) index harnesses satellite technology to create a dynamic picture of forest cover. This sophisticated tool processes multi-temporal imagery, detecting subtle changes in vegetation cover that might escape the naked eye. Interpreting the resulting index maps, which clearly show areas of deforestation, reforestation, and stable forest cover, is a breeze. By providing early warnings of illegal logging or encroachment, it empowers stakeholders to take swift, informed action to protect their assets and the environment.
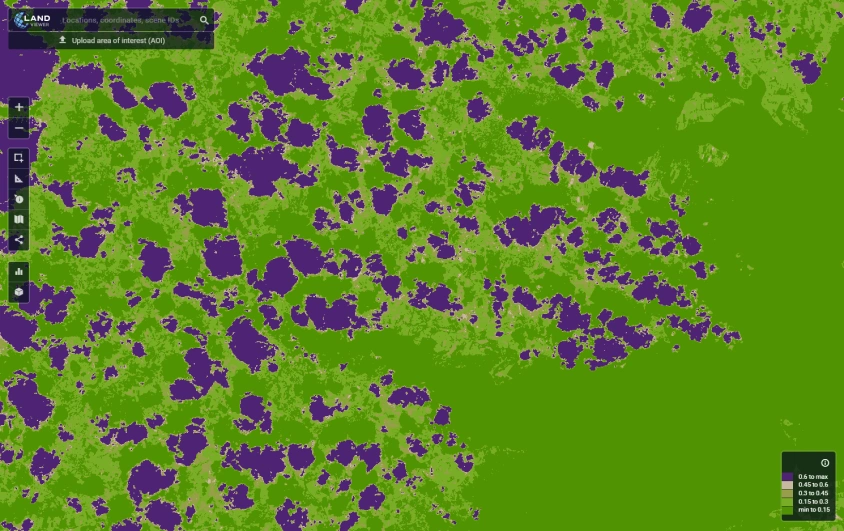
Land/Water Satellite Images
The land/water band composite utilizes specific spectral bands to highlight the contrast between land and water surfaces. By assigning unique colors to different surface types, it creates a visually striking and informative image. To interpret such imagery, remember, that it typically displays:
- land in hues of orange and green;
- ice in vibrant magenta;
- water in hues of blue.
By interpreting land/water satellite images, businesses, governments, and NGOs can precisely monitor shoreline changes, assess flood aftermath, analyze bank erosion, and much more.
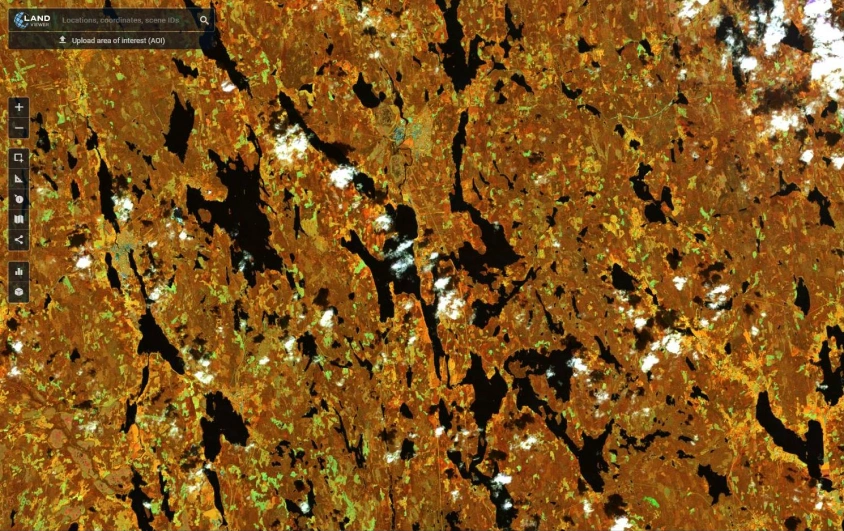
Scene Classification
Scene classification is a powerful technique that automatically categorizes land cover types using advanced algorithms. This process analyzes spectral signatures and spatial patterns to identify distinct features within an image. In the provided example from EOSDA LandViewer, the classification map distinguishes between cloud types, shadows, cloud shadows, plants, soils, water, and snow. Additionally, it generates quality indicators such as cloud and snow probability maps that are quick and simple to interpret.
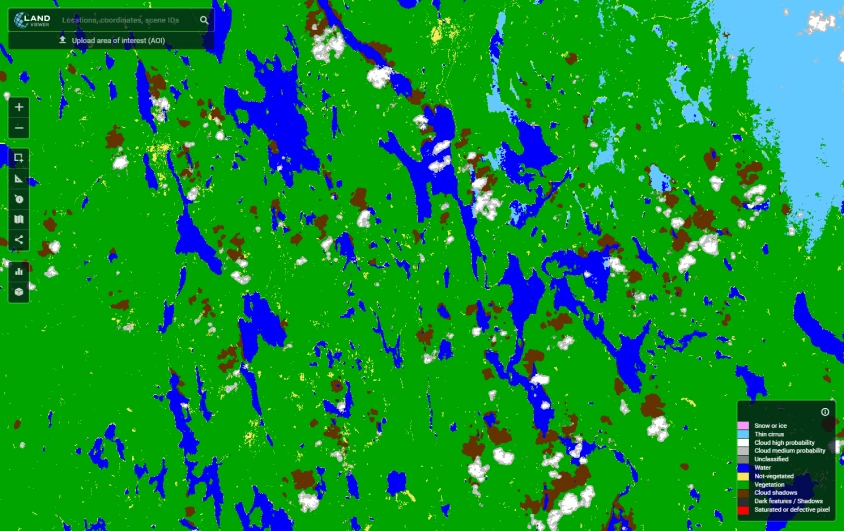
Mastering Satellite Imagery Interpretation: From Pixels To Insights
Interpreting satellite imagery correctly is your window to Earth’s secrets. From true color snapshots revealing land use patterns to false color composites exposing hidden vegetation stress, each band combination unlocks unique insights. Interpreting NDVI maps empowers farmers to optimize crop health, while land/water imagery analysis guides coastal management. EOSDA LandViewer has the power to become your curator, organizing this vast collection for simple exploration. Every pixel tells a story, and our ability to read these space-derived clues might shape the future of Earth.
About the author:
Petro Kogut has a PhD in Physics and Mathematics and is the author of multiple scientific publications. He is the Soros Associated Professor as well as the head of the department of differential equations in the Oles Honchar Dnipro National University and has received a number of grants, prizes, honorary decorations, medals, and other awards. Prof. Dr. Petro Kogut is a science advisor for EOSDA.
Recent articles
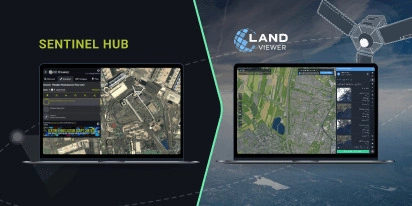
Transition Guide From Sentinel Hub EO Browser To EOSDA LandViewer
Need an EO Browser alternative that’s just as familiar but more flexible? EOSDA LandViewer offers free recent imagery, a vast satellite data archive, and advanced analytical tools.
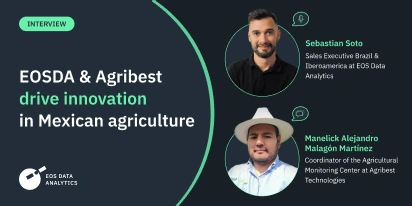
EOSDA & Agribest: Driving Agtech Growth In Mexico
In this insightful interview, Agribest shares how its collaboration with EOSDA is shaping Mexico’s agricultural future, with a focus on profitability, sustainability, and technology.
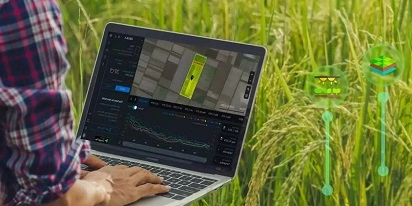
Top Ready-Made Agriculture Tools & Softwares for 2025
Agriculture softwares are silent partners in the field, working tirelessly behind the scenes to support farmers with insights and precision to cultivate sustainable agricultural ecosystems.