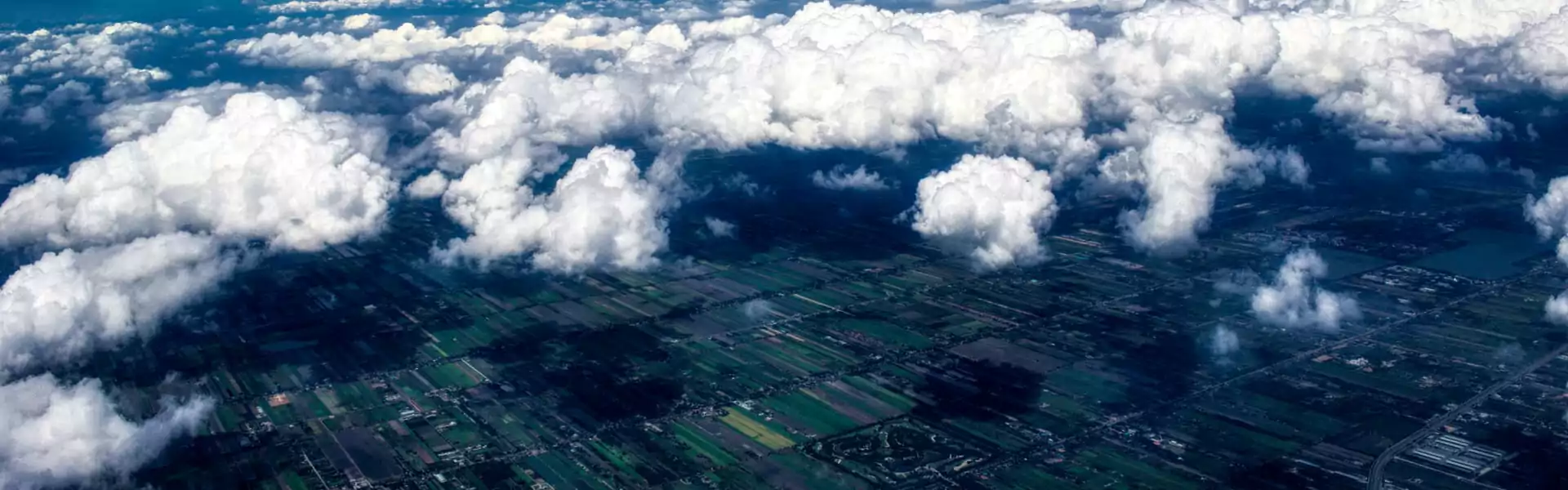
Cloud Mask: What Makes A Difference For Data Accuracy
Satellite imagery benefits multiple industries flaunting a wide range of applications. Yet, it would not be entirely possible without cloud masking techniques. Unlike SAR aperture, optical satellite sensors cannot provide a clear image on a cloudy day, so the issue has to be fixed. This is when cloud masking proves to be an efficient problem solution. This way, the process gets seamless. While satellites observe the situation from the sky, EOSDA computes spatial data with specific algorithms for actionable analytics.
Why Is Cloud Masking Necessary?
Cloud masking in remote sensing prepares imagery for processing and improves product generation.
In preprocessing cloud masking, cloud coverage is not the only trouble. Visibility is also reduced by the shadows they cast and thus decrease the reflectance capability of target objects. Clouds (and, correspondingly, their shadows) differ in shape, size, altitude that depend on the geographic position and climatic peculiarities of the studied region.
Information about clouds is available at multiple sources, unlike information about their shadows, which definitely matters when it comes to image accuracy. Another thing is mask scalability. There should be sufficient image resolution to zoom not only in the entire field but in its separate zones as well. Lacking details leads to errors.
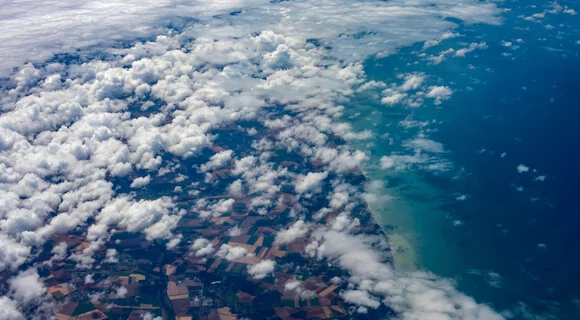
Where Are Clouds-Free Satellite Images Used?
Since unidentified clouds cause analytic errors, the issue requires proper control. Cloud masking advantage is essential for many spheres relying on remote sensing and change tracking, both for governmental and commercial needs. The raster cloud mask of the image series improves analytics for agriculture, forestry, oil & gas, mining, construction, transportation, communications, environmental protection, military and law enforcement, emergency and disaster response, etc. In particular, big data is used to generate additional layers that give extra insights for researchers and businesses.
For example, such layers are incorporated in farming software. They provide growers with the most recent and credible information to make weighted decisions regarding
- rates and time for seeding
- harvesting
- precision irrigation
- fertilization
- pest control
- weed management, etc.
EOSDA LandViewer
Perform satellite mapping of any large area via specialized GIS tools available in one of the largest catalogs of free satellite images.
There are many scenarios to apply the masking technique, depending on the purposes and the task stack. Irrelevant when the analysis of image series takes place, and to get more accurate results, it is necessary:
- to study the image fragment without clouds, or
- to replace the unclear fragments with clear ones whenever possible.
In any case, the issue has a negative impact on the useful data analysis. This is why it is necessary to remove them from the analysis process to reduce the error possibility in the estimated results.
Does It Make Sense To Separate Clouds From Haze?
The standard classification distinguishes clouds and haze as separate categories, yet it is not quite justified. These are similar objects, which differ by atmospheric relative humidity. The concentration of water vapor is higher in clouds and lower in haze. For this reason, it may be hard to separate one from the other – their borderlines can be obscure.
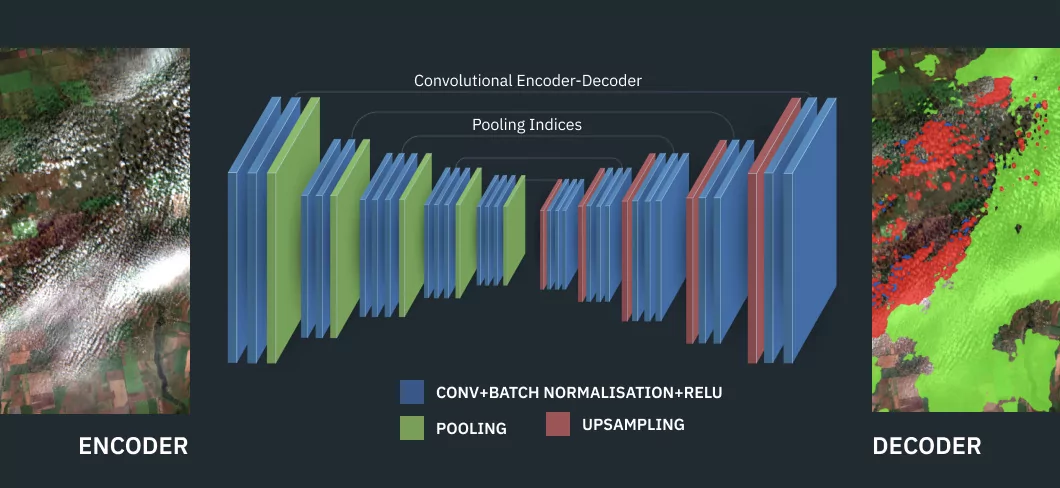
Cloud Mask Implementation In EOSDA Crop Monitoring
In the EOSDA Crop Monitoring tool, masks allow removing useless data that could negatively affect the analysis results.
However, it would be wrong to consider that cloud detection is the same as cloud masking. These are two different processes. Detection aims at identifying clouds in the image and obtaining a mask. Masking is the next step. It uses the received mask to “hide” useless data, for example, while generating indices in the product.
With a precise mask, EOSDA enables its customers to analyze satellite images more accurately thanks to excluding images with 60% cloudiness and more, as too dense cover in the image can decrease the quality of the obtained results.
Also, there is a cloud-free NDVI in EOSDA Crop Monitoring that uses radar satellite data.
Enjoy The Difference
The EOSDA scientists calculate multiple indices, and clouded images are undesirable for all of them. So, the best option is to remove unsuitable imagery from the analysis for more accuracy.
As appears, it is also important to differentiate between deep shadows and dense clouds as well as light shadows and haze. Furthermore, it is possible to ignore haze for some tasks, but it won’t work for others.
The point is to be flexible and adjust the results to the customer’s needs, and EOSDA does it. Enjoy extra accuracy with the innovative approach and keep ahead of the game.
About the author:
Kateryna Sergieieva has a Ph.D. in information technologies and 15 years of experience in remote sensing. She is a Senior Scientist at EOSDA responsible for developing technologies for satellite monitoring and surface feature change detection. Kateryna is an author of over 60 scientific publications.
Recent articles
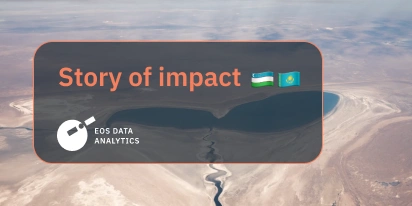
The Aral Sea’s Rebirth: Hope Blooms
This story of impact explores the Aral Sea's restoration, highlighting EOS Data Analytics' satellite insights, and showcasing collaborative efforts to revive a vital ecosystem.
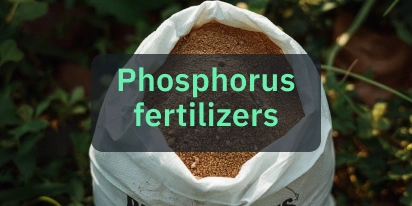
Phosphorus Fertilizers: Types And Role In Agriculture
Key to optimizing crop yields and quality, phosphorus fertilizers are indispensable in agriculture. Explore their types, application methods, and environmental impact in our comprehensive overview.
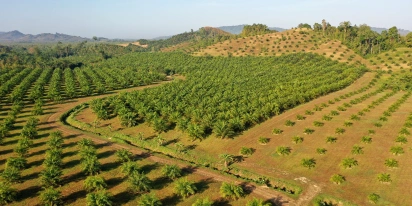
Oil Palm Farming In Southeast Asia: Sustainability Path
Oil palm cultivation in Indonesia, Malaysia, and Thailand forms the foundation of their economies. But plenty of related environmental issues force producers to drastically change their approaches.