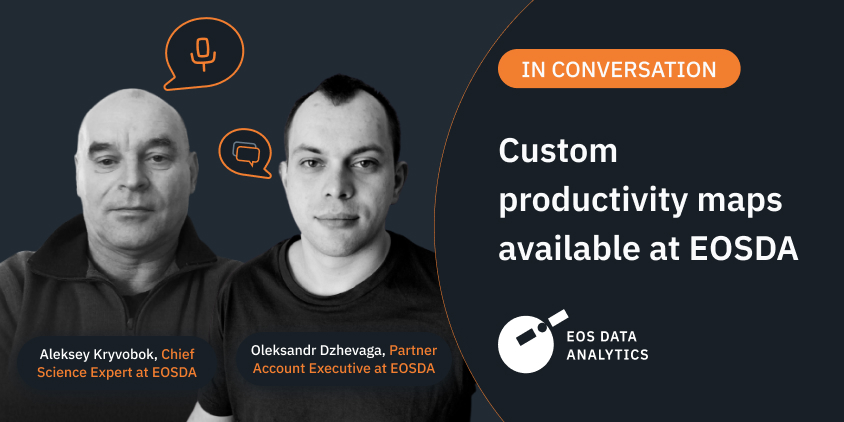
EOSDA Aids Higher Crop Yields With Productivity Maps
For agricultural businesses, reliable tracking of crop productivity is crucial for optimizing operations, managing supply chains, and complying with regulations. Research indicates that yields for high-demand crops like wheat have steadily increased since the mid-20th century . However, statistics also reveal an increase in fertilizer use, which could lead to soil erosion and a decrease in soil lifespan. In fact, 16% of soils have a lifespan of less than 100 years, meaning that some regions may only have roughly 100 yields left before the land becomes infertile . This highlights the importance of sustainable farming practices that aim to balance increased productivity with long-term soil health and fertility.
Remote sensing analytics might help to visualize and compare crop productivity and its contributing factors. We spoke to Oleksandr Dzhevaga, EOSDA’s Partner Account Executive, and Aleksey Kryvobok, Chief Science Expert at EOS Data Analytics, about a new custom solution that they’ve been working on, called Productivity Maps based on Combined Productivity Index.
Why Would Agrarians Need AI-Powered Tools For Assessing Crop Productivity?
Oleksandr Dzhevaga: We are seeing a growing demand for streamlined analytics in agriculture for various reasons. For one, due to the EU’s Farm to Fork Strategy, the loss of nutrients should be reduced by 50% until 2030, and the use of fertilizers should be decreased by 20% while preserving soil fertility . This strategy results in a number of directives that are enforceable for all agricultural businesses within the EU. Furthermore, different factors, from climate change to import-export balance require growers to seek instruments that will help them preserve productivity in a healthy and cost-efficient way.
Some of the objectives of AI-powered tools for agriculture are:
- To make predictions on crop state.
- To enable quick analysis of data and make actionable recommendations.
- To optimize irrigation schedules, fertilizer application, and other critical farm operations that impact crop productivity.
- To detect and predict pests and infestations in crops.
- To mitigate weather risks and have an understanding of soil moisture, temperature, humidity, precipitation, etc. to make informed decisions.
As you can see, the use of AI-powered tools gives advanced capabilities to assess crop productivity and health with greater accuracy and efficiency. The use of advanced analytics facilitates yield optimization, risk mitigation, and data-driven decisions to enhance agricultural practices.
What Are The Productivity Maps Based On Combined Productivity Index As A Custom Solution In EOSDA?
Aleksey Kryvobok: Productivity Maps based on Combined Productivity Index (further – PMs) are generated using input data combined from sources of different natures, like satellite imagery and biophysical models. PMs allow us to find out in which parts of the field the productivity (vegetation) was consistently high, and in which, it was consistently low.
The output is a map, enriched with visualization based on the NDVI (Normalized Difference Vegetation Index) along with the results of biophysical modeling. The latter consider several additional factors (crop type, weather conditions, soil type, topography, etc.). This allows for a more accurate analysis since more variables are taken into consideration for deriving the output value. In addition, the Combined Productivity Index can be used to assess the specifics of each crop separately, that is, the user can have different productivity maps for different crops.
Oleksandr Dzhevaga: Yes, our clients have been expressing interest in the development of such diversified analytics. It is very important since each crop type requires a specific set of conditions for producing a good harvest.
Satellite Data Comes In Numbers/Pixels And Crops Are Measured In Weight Units. What Units Of Measurement Do You Use For Assessing Productivity?
Aleksey Kryvobok: The productivity index is a value without units of measurement. This is related to the fact that our methodology combines two different data sources with different units of measurement:
- Productivity index is calculated based on satellite images (namely based on vegetation indices) that take a value from 0 to 1.
- Crop-modeled productivity, based on the results of biophysical modeling in units like kg/ha.
The final (combined) index is the value obtained from biophysical modeling, multiplied by the productivity index calculated from satellite data.
Therefore, this productivity index doesn’t have specific units of measurement. We can somehow compare the productivity index value with kg/ha, but this will not be completely correct. The best way to use the index is to compare the productivity of different areas of interest (AOIs) across a unified scale, which is called clustering. Then, by estimating the obtained results, one can understand which zone is more productive and which one is less productive (given several parameters mentioned above).
To better understand this, let’s refer to an example (maize):
- Productivity index based on satellite data for pixel/ zone = 0.8
- Result of biophysical modeling = 10,000 (supposedly kg/ha)
- Final weighted (combined) productivity Index pixel/ zone = 8,000 (without units of measurement)
What Is Clustering In Productivity Maps And How Can It Be Used?
Oleksandr Dzhevaga: In the raw version (without additional post-processing), Productivity Maps are georeferenced images, consisting of sets of pixels, each pixel containing the value of the Combined Productivity Index. This is not always a convenient format for using such data, especially when it needs to be used for machinery or high-level analysis of a large area.
In order to make the use of productivity maps more convenient for the purposes mentioned above, clustering (or zoning) is usually carried out. Clustering is dividing the productivity map into different homogeneous zones, depending on the pixel index values. This means that pixels with similar index values are combined into one zone, i.e., cluster.
Aleksey Kryvobok: We can choose a different number of clusters for different cases. For example, you can select 5 clusters (very high productivity, high productivity, average productivity, moderate productivity, and low productivity). Then, for each cluster, choose an interval of index values. And if the index value in some pixel falls within the selected interval, then the pixel will be assigned to a cluster, like in the image below.
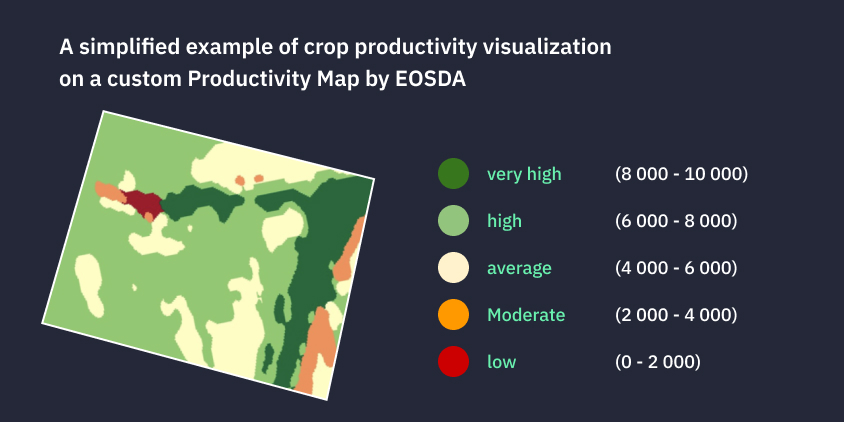
Aleksey Kryvobok: Further, such clusters can be more conveniently used, for example, for differentiated fertilization. The clustered productivity map is loaded into the machine, and the fertilizer rate is set for each class (for example, the highest fertilization rate is set for the low productivity cluster, and the lowest rate for the very high productivity cluster). The machine uses navigation and georeferences of a productivity map, so when the machine enters, let’s say, a low productivity zone, the amount of fertilizer dropped will increase, and so on, depending on the zone in which the machine is located.
AI Models Are Known For Being Brittle. If A Model Is Trained On One AOI, It Can Be Erroneous On Another One. How Do You Ensure Accuracy Across Different AOIs?
Aleksey Kryvobok: The Combined Productivity Index is based on two components: the results of calculations of the vegetation index (NDVI) and the results of biophysical modeling. To train a custom model for a PM, we need to train separate models for each of these two components and then combine the results.
As for the first component, everything is quite simple here. The index is calculated based on multispectral satellite images, which capture a specific wavelength reflection from the surface of the Earth. With a change in the features of the earth’s surface (for example, in another region), these spectral reflective characteristics change, and the value of the indices also changes. Equations and approaches for calculating indices have been developed long ago and validated according to various criteria by many communities of scientists.
Oleksandr Dzhevaga: However, the situation is slightly different with the second component (biophysical modeling). It uses a well-known method, the WOFOST model, which has been adapted by EOSDA scientists for the purpose of calculating productivity maps based on the Combined Productivity Index. With the help of our variation of this dynamic model, we can estimate the untapped crop potential, enriched by knowledge of environmental and climatic conditions.
Aleksey Kryvobok: This becomes possible because the input parameters of the model are local data on soil types, weather, and features of crop development (phenological data) in a particular region. This means the model will receive specific parameters inherent to a particular location as input. For example, phenological data are studied separately for each agro-climatic region by the EOSDA R&D team. This allows us to refine the algorithm so that it takes into account the specifics of the region for which the analysis is carried out.
The model can also be validated and calibrated further if reliable ground data is available in selected regions, such as weather data from ground-based weather stations, actual crop yield data, high spatial resolution soil maps, etc.
How To Order Productivity Maps From The EOSDA Team?
Aleksey Kryvobok: We need to obtain certain data to start training the AI model. In the case of Productivity Maps based on the Combined Productivity Index, it’d be:
- Area of interest: To check the quality and acceptability of the simulation, it is proposed to provide AOIs from at least two regions with different climatic and soil characteristics.
- Key crops of interest and phenological data on target crops for AOI (if available)
- Soil type data for AOI (if available)
- Terrain data for AOI (if available)
- Weather historical data (if available)
Oleksandr Dzhevaga: Such Productivity Maps are custom projects and every custom project is unique. Usually, agrarians that express interest in training a model, contact us and together we discuss how we can implement their project in accordance with the requirements. All of our custom solution developments are carried out by our team of scientists which includes doctors and professors in a total of 60 in-house data scientists and GIS specialists. After the initial discussion, a detailed proposal is shared with the customer to provide the estimates, duration, and expected results of the project.
What Types Of Work Can Such Custom Projects Include?
Oleksandr Dzhevaga: Let me highlight some of the potential tasks that we can cover in a custom Productivity Mapping project.
- Refining the automated pipelines (biophysical modeling, weather data, etc.)
- Analyzing vegetation and climatic features of the client’s unique area of interest
- Launching and monitoring the operation of pipelines, e.g. searching, downloading, and combining satellite images, calculating the productivity index based on satellite data
- Biophysical modeling for target crops for the required area and historical period
- Combining the results of calculations and obtaining a combined productivity index
- Post-processing the result, search, and correction of anomalies (if any), data rasterization, data clustering, and visualization
- Generating reports on the model training results.
These are just some of the examples of what we do that come up frequently in our projects. Yet, the potential of a custom PM can be extended even further.
Can You Name Some Of The Recent Successful Use Cases For The Customers? What Were The Customers’ Goals And How Did We Meet Them?
Oleksandr Dzhevaga: Recently we managed to successfully deliver a PoC for productivity maps for a major international ag science and technology company in the US. We had the opportunity to directly engage with their R&D team and develop the Productivity Maps based on their stated requirements.
Aleksey Kryvobok: The main goal of the project was to confirm the high quality and accuracy of EOSDA solutions regarding PMs generated using a combined approach based on satellite data and biophysical model data. The output result successfully passed the internal validation process conducted by the client, which is especially valuable given the scale of their business.
So, When The AI Model For Custom Productivity Maps Is Trained, What’s Next? How Can A Customer Implement It In Their Own Work Processes And Software?
Aleksey Kryvobok: This depends on the format of the result. PMs can be provided for the selected area of interest in two main formats:
- Vector mask, which will divide the AOI into productivity zones according to the productivity index values. For more accurate data, the area will most likely be divided into smaller divisions (fields / sections) within which zones will be displayed.
- Raster mask, with per pixel display of the productivity index value. These indices for each pixel can be extracted for further analysis, if necessary.
Oleksandr Dzhevaga: The client can choose the desired data format and either display the results with their software (and for example, consult their clients) or use this data for further experiments and developments (since we provide very detailed per-pixel data and detailed instructions for correctly interpreting the data).
What Is The Competitive Advantage Of Such Productivity Maps From EOSDA?
Oleksandr Dzhevaga: Competitive advantage on a business level can be understood with the main areas of application of Productivity Maps:
- PM can be used for differential seeding. For example, more seeds can be sown in a more productive zone, and fewer seeds – in a less productive zone, to get the maximum yield.
- Also, with its help, farmers can define areas for sampling soil. Soil sampling is needed for optimizing plant growth, and can be done in different ways. Having a custom PM allows one to take a smaller number of samples due to extracting them not along the grid, but from zones with different productivity. This method is more accurate and cost-effective.
- Such maps are used to apply potash and phosphorus fertilizers. These fertilizers need to be applied less frequently than Nitrogen fertilizers (depending on soil composition, climate, etc.), because they take longer to be washed out of the soil, thus, the field analysis is compiled over longer iterations.
- PM allows us to perform analytics on the potential of certain territories in terms of productivity for different types of crops. This is an important tool for agricultural enterprises that analyze the feasibility of investments and entry into different territories to extend business operations.
Aleksey Kryvobok: When it comes to the algorithmic part of our model, our advantage is that we train the model on two or more cross-validating datasets, which makes it come to more accurate analytical conclusions.
Furthermore, the maturity of our technology is definitely something that lets us outrun the competition. Our data scientists are seasoned neural network creators who can shape mathematical models beyond conventional approaches known on the market. Those models are also enriched with the expertise of GIS scientists, soil scientists, and phenology experts.
By the way, our newest research findings are registered with the United States Patent and Trademark Office, which is solid proof of our science team’s exceptional performance. Namely, one of our registered discoveries is used in EOSDA Crop Monitoring.
The fact that we can tailor neural nets according to our customer’s needs is why we call PMs custom projects. Essentially, every other model can turn into something new. On top of that, it will be reproducible – we can help smooth out the code and processes so that a custom PM brings value continuously. It’s not just a one-time thing.
Ok, So EOSDA Has Productivity Maps As A Custom Solution And Productivity Maps In EOSDA Crop Monitoring. What Is The Difference Between The Two?
Aleksey Kryvobok: Productivity Maps as a custom solution is based on Combined Productivity Index, which includes vegetation index data derived from satellite imagery, as well as biophysical modeling data. To create custom PMs, we need to adapt the biophysical model to run for a specific geographical location (climate data, soil, crop parameters, etc.) that is related to each specific case. Whereas Productivity Maps as a feature of the EOSDA Crop Monitoring platform automatically calculates an average of the highest NDVI values over a period of time, specified by the user. This operation takes only up to a minute. All in all, a Productivity Maps feature on a platform is a quick solution that everyone can use for variable rate application (inputs and seeds), as well as defining locations for soil sampling. Whereas PMs as the custom solution are more precise since more parameters are considered, but they take time and human expertise to make.
Got It! Finally, What Are EOSDA’s Future Plans For The Growth Of Custom Productivity Maps?
Aleksey Kryvobok: Following our successful Proof of Concepts, we’re confident that our solution is both viable and scalable. We have developed a set of pipeline methodologies that allow our team to efficiently calculate the combined productivity index for large areas. Therefore, our nearest plans include training custom models on larger areas than during the PoC process, plus working with biophysical modeling more extensively.
Oleksandr Dzhevaga: There is also a potential for increasing the accuracy of modeling, in particular, due to the following factors:
- Yield statistics: If a customer can provide historical data on yields in the regions of interest, then this could refine the model (due to more reliable model parameters) and also bring units closer to kg/ha (but they will not be exactly the same in any case).
- Weather data: Using a high-resolution weather grid, and even better data from ground stations (if available from the customer), we can improve the accuracy and equability of the results.
- Data on soil types and topography: These are also factors that can improve accuracy if locally precise high-resolution digitized soil and terrain maps are available. As an alternative, EOSDA could potentially improve the spatial resolution of existing soil maps.
- Other data: Any other available reliable ground truth data that can become additional model parameters.
Aleksey Kryvobok: That’s right because our approach is based on biophysical models and even if it’s something like an exotic plant and we have data about it (phenology and crop parameters), we can work with that. The developed methodology allows EOS Data Analytics to expand the list of supported crops if necessary, whether it’s common crops or rare ones.
About the author:
Rim Elijah holds a double degree in business administration and political science from Stockholm University. As a VP of Sales at EOSDA, she oversees all aspects of business model development and implementation and the growth of the company’s global coverage. She has successfully established a number of strategic partnerships with an emphasis on sustainable solutions in Africa and Asia.
Recent articles
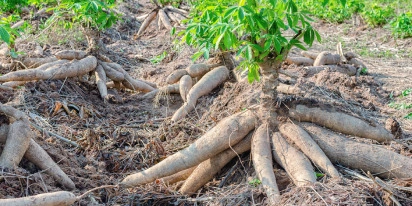
Growing Cassava: From Land Choice & Planting To Harvest
Growing cassava can work well even in poor soils and dry conditions, unsuitable for most other crops. So, farmers can achieve high yields even under less-than-ideal conditions without too much input.
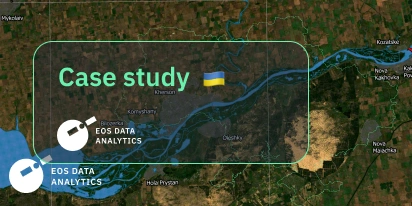
Kakhovka Dam Flooding Detection In Ukraine For PEJ
EOSDA helped Project Expedite Justice calculate the flooding of the surrounding area around the Kakhovka Dam destruction on June 6, 2023, for the justice report for the International Criminal Court.
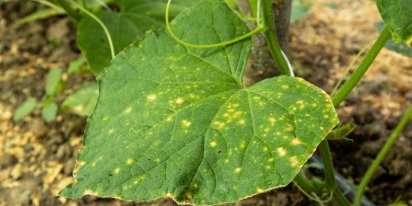
Downy Mildew: How To Identify, Prevent, And Control
Controlling downy mildew — an aggressive crop disease — requires early identification and action. Targeted prevention strategies have a direct impact on the overall economic sustainability of farming.