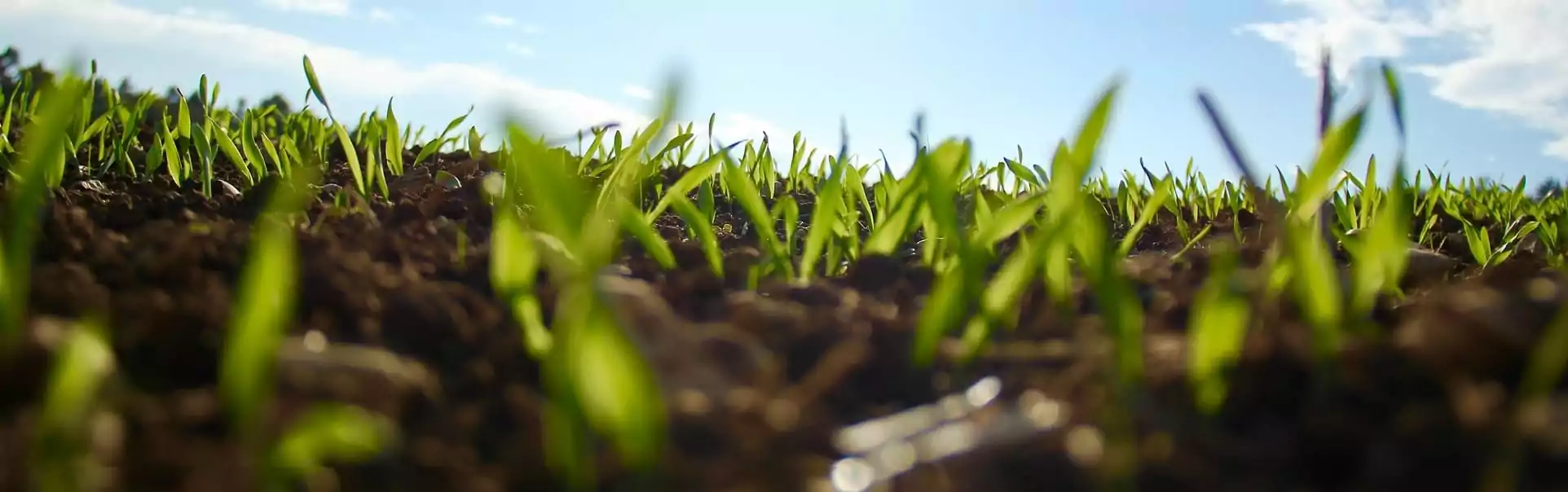
Vegetation Indices To Drive Digital Agri Solutions
Vegetation indices are an important parameter of crop development analytics. Among numerous advantages of vegetation indices in remote sensing, data precision and miles-away control are the key drivers to adopt the handy technology.
As sensors advance, Earth-observing satellites provide remote sensing experts with new data to fuel their research and improve the existing analysis. By embracing the popping up novelties in spectral vegetation indices applications, the companies that already have their index-based software, as well as those who are planning to launch a new one, can significantly boost the demand for their agriculture-related products.
Vegetation Index For Agriculture In Digital Solutions
Integration of satellite-based analytics can increase the current value of farming apps, as well as resolve their certain present limitations. In particular, by incorporating vegetation indices into their products, software providers can unlock and enjoy the following benefits for their business:
- availability of high-level analysis;
- possibility to expand their service;
- access to different images sources in one place;
- reduction of internal expenses;
- additional value of their products.
Numerous advantages of vegetation indices in remote sensing also help improve customer experience. Satellite imagery vs. other aerial data allow:
- saving costs on drone operations, data processing, and interpretation;
- covering larger territories compared to aerial imagery;
- reducing expenses for field inspection: additional UAV observations are more costly than regular satellite revisits;
- getting data analytics within a shorter time in a preferred format;
- monitoring fields irrespective of wind gusts.
With satellite images, agri software providers can significantly expand their existing pool of aerial data, as well as save their time and resources, while end-users can access more data faster. Thus, vegetation indices in remote sensing are useful in the distant high-level analysis of the crop state. If any issue arises, farmers can double-check only the spotted areas instead of inspecting the whole field.
Vegetation Indices Available On EOSDA Crop Monitoring By Default
Our standard crop analytics comprises NDVI, ReCI, NDRE, and MSAVI, among the many more possibilities on request.
All default vegetation indices are also included in the white label. Furthermore, our white label product also comprises an extended array of other data like soil moisture, weather analytics, and the whole stack of standard EOSDA Crop Monitoring features.
Our white label customers can host the platform on their own domain and use the data under their logo and brand. EOSDA carries out complete white label product launch and technical support, including the company’s expertise in satellite-based analysis.
EOSDA Crop Monitoring
Access high-resolution satellite images to ensure effective fields management!
Normalized Difference Vegetation Index (NDVI)
Among the typical spectral vegetation indices, NDVI is one of the most suitable to track crop development dynamics since it measures photosynthetically active biomass in plants. However, this vegetation index is quite sensitive to soil brightness and atmospheric effects, mitigated in other indices like EVI, SAVI, ARVI, GCL, or SIPI.
Formula: NDVI = (NIR – RED) / (NIR + RED)
Key fact: NDVI is the most common vegetation index in remote sensing. It can be used throughout the whole crop production season except when vegetation cover is too scarce, so its spectral reflectance is too low.
When to use: NDVI values are the most accurate in the middle of the season at the stage of active crop growth.
Red-Edge Chlorophyll Vegetation Index (RECl)
The ReCI vegetation index is responsive to chlorophyll content in leaves that is nourished by nitrogen. ReCI shows the photosynthetic activity of the canopy cover.
Formula: ReCI = (NIR / RED) – 1
Key fact: Because chlorophyll content directly depends on nitrogen level in plants, responsible for their “greenness”, this vegetation index in remote sensing helps detect areas with yellow or shed foliage.
When to use: ReCI values are most useful at the stage of active vegetation development but are not suitable for the season of harvesting.
Normalized Difference Red Edge Vegetation Index (NDRE)
The NDRE index combines the Near-infrared (NIR) spectral bands and a specific band for the narrow range between the visible red and the red-NIR transition zone (the so-called red-edge region). For the best data precision, it is recommended to use NDRE in combination with NDVI.
Formula: NDRE = (NIR – RED EDGE) / (NIR + RED EDGE)
Key fact: The given vegetation index applies for high-density canopy cover.
When to use: NDRE is typically used to monitor crops that have reached the maturity stage.
Modified Soil-Adjusted Vegetation Index (MSAVI)
The MSAVI vegetation index is designated to mitigate soil effects on EOSDA Crop Monitoring results. Therefore, it is applied when NDVI can’t provide accurate values, particularly, with a high percentage of bare soil, scarce vegetation, or low chlorophyll content in plants.
Formula: MSAVI = (2 * Band 4 + 1 – sqrt ((2 * Band 4 + 1)2 – 8 * (Band 4 – Band 3))) / 2
Key fact: Since MSAVI is adjusted to soil effects and is sensitive to early vegetation in the field, it works even when the earth is hardly covered with crops.
When to use: MSAVI is useful at the very beginning of crop production season – when seedlings start to establish.
Vegetation Indices Available On EOSDA Crop Monitoring By Request
If a client already has their own resources and doesn’t need a turnkey solution, they still can augment their product through API. Access via API to vegetation indices is not limited to the four default indices on EOSDA Crop Monitoring. These also include a comprehensive set of GNDVI, NDWI, SAVI, OSAVI, ARVI, EVI, VARI, LAI, NBR, SIPI, GCI, NDSI, ISTACK, as well as any other custom indices needed.
Green Normalized Difference Vegetation Index (GNDVI)
The GNDVI index is a modification of NDVI and it also uses near-infrared but substitutes VIS green for VIS red (540 to 570 nm).
Formula: GNDVI = (NIR – GREEN) / (NIR + GREEN)
Key fact: GNDVI measures chlorophyll content more accurately than NDVI.
When to use: to detect wilted or aging crops and to measure nitrogen content in leaves when an extreme red channel is not available, monitor vegetation with dense canopies or at maturity stages.
Normalized Difference Water Index (NDWI)
The NDWI was initially elaborated to outline open water bodies and assess their turbidity, mitigating the reflectance of soil and land vegetation cover. NDWI is retrieved with a near-infrared and visible green band combination.
Formula: NDWI = (GREEN – NIR) / (GREEN + NIR)
Key facts: The NDWI index is often confused with NDMI (Normalized Difference Moisture Index). NDWI uses SWIR (Short Wave Infrared) and NIR channels. NIR reflectance allows analyzing dry matter content in vegetation foliage and internal leaf structure, while SWIR reflectance shows the changes in plant water content and mesophyll structure. When combined, NIR and SWIR bands give a better idea of plant water content because the water in the internal leaf structure impacts the spectral reflectance in SWIR.
When to use: detection of flooded agricultural lands; allocation of flooding on the field; detection irrigated farmland; allocation of wetlands.
Soil Adjusted Vegetation Index (SAVI)
The SAVI was introduced to mitigate the impact of soil brightness. Its creator Huete added a soil adjustment factor L to the equation of NDVI in order to correct for soil noise effects (soil color, soil moisture, soil variability across regions, etc.), which tend to impact the results.
Formula: SAVI = ((NIR – RED) / (NIR + RED + L)) * (1 + L)
Key fact: L varies from –1 to +1, depending on the green vegetation density in the questioned area. In areas with high green vegetation L= 0, and in this case, SAVI is the same as NDVI. Conversely, L = 1 for low green vegetation zones. Most typically, L is set to 0.5 to adjust to most land cover .
When to use: for analysis of young crops; for arid regions with sparse vegetation (less than 15% of total area) and exposed soil surfaces.
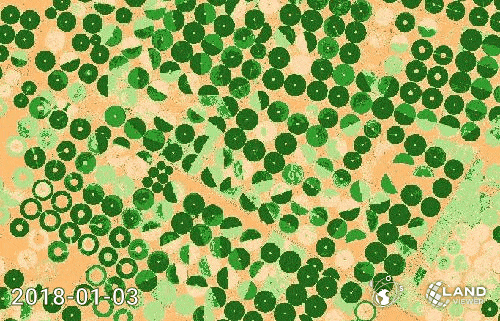
Optimized Soil Adjusted Vegetation Index (OSAVI)
The OSAVI vegetation index is a modified SAVI and also uses reflectance in the NIR and red spectrum. The difference between the two indices is that OSAVI takes into account the standard value of the canopy background adjustment factor (0.16).
Formula: OSAVI = (NIR – RED) / (NIR + RED + 0.16)
Key fact: The adjustment allows greater soil variation in OSAVI compared to SAVI when canopy cover is low. OSAVI has a better sensitivity to canopy cover exceeding 50%.
Where to use: to monitor areas with low-density vegetation with bare soil areas through the canopy.
Atmospherically Resistant Vegetation Index (ARVI)
This is the first vegetation index, relatively insensitive to atmospheric factors (e.g., aerosols). As the formula shows, Kaufman and Tanré corrected NDVI to mitigate atmospheric scattering effects by doubling the red spectrum measurements and adding blue wavelengths.
Formula: ARVI = (NIR – (2 * RED) + BLUE) / (NIR + (2 * RED) + BLUE)
Key fact: Compared to other indices, ARVI is also more insensitive to relief effects, being particularly useful to monitor tropical mountainous regions often covered with soot due to slash-and-burn agriculture.
When to use: for regions with high content of atmospheric aerosol (e.g. rain, fog, dust, smoke, air pollution).
Enhanced Vegetation Index (EVI)
Liu and Huete introduced the EVI vegetation index to adjust NDVI results to atmospheric and soil noises, particularly in dense vegetation areas, as well as to mitigate saturation in most cases. The value range for EVI is –1 to +1, and for healthy vegetation, it varies between 0.2 and 0.8.
Formula: EVI = 2.5 * ((NIR – RED) / ((NIR) + (C1 * RED) – (C2 * BLUE) + L))
Key fact: EVI contains coefficients C1 and C2 to correct for aerosol scattering present in the atmosphere, and L to adjust for soil and canopy background. Beginner GIS analysts may be confused by what values should be used and how to calculate EVI for different satellite data. Traditionally, for NASA’s MODIS sensor (which the EVI vegetation index was developed for) C1=6, C2=7.5, and L=1. In case you’re wondering how to see Enhanced Vegetation Index using Sentinel 2 or Landsat 8 data, use the same values or simply use EOSDA Crop Monitoring, which also allows downloading the results.
When to use: for analyzing areas of Earth with large amounts of chlorophyll (such as rainforests), and preferably with minimum topographic effects (non-mountainous regions).
Visible Atmospherically Resistant Index (VARI)
The VARI index is perfect for RGB or color images since it works with the whole visible segment of the electromagnetic spectrum (comprising red, green, and blue color bands). Its specific task is to enhance vegetation under strong atmospheric impact while smoothing illumination variations. VARI can be used for the following satellite sensors: Sentinel-2, Landsat-8, GeoEye-1, Pleiades-1, Quickbird, and IKONOS.
Formula: VARI = (GREEN – RED) / (GREEN + RED – BLUE)
Key fact: Thanks to low sensitivity to atmospheric impact, the error of VARI for vegetation monitoring in conditions of different atmospheric thickness is less than 10%.
When to use: crop state assessment when minimum sensitivity to atmospheric effects is required.
Leaf Area Vegetation Index (LAI)
The LAI index is designed to analyze the foliage surface of our planet and it estimates the quantity of leaves in a specific region. LAI is a unitless measure that is calculated as the ratio of the one-sided (illuminated) foliage area to the soil surface it can cover. This vegetation index is important to monitor crop and forest health, the environment, and climatic conditions. LAI is scalable to an individual plant, crop(s) in the field, or the whole region.
Formula: LAI = leaf area (m2) / ground area (m2)
Key Facts: The LAI leaf vegetation index in remote sensing was introduced for the NASA MODIS sensor to improve NDVI data. Unlike the latter, it takes into account topographic features, and the spectral bands that are used for its calculations undergo atmospheric correction.
If LAI = 3, then the leaves can cover the surface threefold. LAI is considered high at 0-3.5. However, its values saturate with clouds and bright objects that should be masked for data accuracy.
When to use: in vegetation health assessment, as input data in a productivity forecasting model.
Normalized Burn Ratio (NBR)
By definition, the Normalized Burn Ratio is used to highlight burned areas following the fire. The equation of the NBR vegetation index includes measurements at both NIR and SWIR wavelengths: healthy vegetation shows high reflectance in the NIR spectrum, whereas the recently burned areas of vegetation reflect highly in the SWIR spectrum.
This vegetation index calculation is based on a raster image with NIR and SWIR bands, e.g., from Landsat-7, Landsat-8, or MODIS. The range of values is between +1 and –1.
Formula: NBR = (NIR – SWIR) / (NIR + SWIR)
Key fact: The NBR fire index has become especially instrumental in the past years as extreme weather conditions cause a significant increase in recent wildfires destroying forest biomass.
When to use: the typical use of NBR for agriculture and forestry is the detection of active wildfires, analysis of burn severity, and monitoring of vegetation survival after the burn.
Structure Intensive Pigment Vegetation Index (SIPI)
The SIPI vegetation index is good for the analysis of vegetation with the variable canopy structure. It estimates the ratio of carotenoids to chlorophyll: the increased value signals vegetation stress.
Formula: SIPI = (NIR – BLUE) / (NIR – RED)
Key fact: Increased SIPI values (high carotenoids and low chlorophyll) may mean crop disease, often inducing chlorophyll loss in vegetation.
When to use: for monitoring plant health in areas with high variability in canopy structure or LAI, for identifying early signs of crop diseases or other causes of stress.
Green Chlorophyll Vegetation Index (GCI)
In remote sensing, the GCI vegetation index is used to estimate the content of leaf chlorophyll in various species of plants. The chlorophyll content reflects the physiological state of vegetation; it decreases in stressed plants and can therefore be used as a measurement of vegetation health.
Formula: GCI = NIR / GREEN – 1
Key fact: Better prediction of chlorophyll amount with the GCI vegetation index can be achieved by using satellite sensors that have broad NIR and green wavelengths.
When to use: to monitor the impact of seasonality, environmental stresses, or applied pesticides on vegetation health.
Normalized Difference Snow Index (NDSI)
The NDSI vegetation index applies to detect snow cover with visible (VIS) green and SWIR or NIR band combinations. The band choice is explained with high snow reflectance in SWIR and low reflectance in VIS, while cloud reflectance in these spectral regions is high. This property allows distinguishing between clouds and snow. The presence of snow is understood through the ratio of the difference in VIS and SWIR reflectance.
Formula: NDSI = (GREEN – SWIR1) / (GREEN + SWIR1)
Key fact: NDSI is similar to Fractional Snow Cover (FSC) in snow detection, but it provides more accurate data than the latter.
When to use: in snow mapping, to differentiate between snow and clouds.
Index Stack ISTACK
This is a combination of three indices (NDVI, NDWI, and NDSI) to generate an image stack. In this merged image stack, a specific landscape is allocated its particular conventional color:
- vegetation is green;
- earth, rocks, bare soil appear in blue;
- snow, clouds, ice, and water all together are attributed purple hues.
Formula: It is a combination of NDSI, NDVI, and NDWI, so the formula depends on a satellite the data is derived from.
Key fact: The stack of indices automatically distinguishes various landscape features, as well as enables image classification and quantitative analysis with index values. By combining various vegetation indices, one can increase the potential of this analysis type to nearly unlimited. However, ISTACK has a drawback: similar hues of snow, clouds, ice, and water complicate their separation.
When to use: automatic differentiation of diverse landscape features with NDVI, NDWI, and NDSI to generate an image stack for Landsat-4,5,7; Sentinel-2; MODIS imagery.
Custom Vegetation Indices
EOSDA Crop Monitoring capabilities go far beyond the above-listed indices available by default and on request. We can tailor the indices specifically for your needs. For example, you can change the NDVI palette or customize it for a particular crop like “NDVI Pulses” and “NDVI Cotton” or whatever crop grown.
A custom solution is a perfect choice if customers:
- need particular spectral vegetation indices for EOSDA Crop Monitoring;
- require to change current indices to match their climate specifics;
- use a specific vegetation index formula;
- prefer individual class thresholds and colors for crop classification;
- want to add another vegetation index for agriculture.
Unlock Your Business Opportunities With EOSDA Crop Monitoring
Among over a hundred various vegetation indices, agricultural software should include an extended stack of the most relevant ones that all will be handy to use in one place. EOSDA provides the necessary analytics to integrate both into the existing and upcoming software for agriculture.
Explore ag solutions for IT companies and developers by EOSDA. Our sales department will advise you on the perfect option to match your or your customers’ needs. Whether you are going to use our product via API, white-label, or custom solutions, you will get accurate and reliable data retrieved with remote sensing and advanced satellite technologies.
Providing precise data-driven analytics globally, EOSDA has earned many happy customers thanks to its deep expertise and rich experience. Reach out to our sales team at sales@eosda.com for details and possibilities of remote sensing applications in your niche.
About the author:
Kateryna Sergieieva has a Ph.D. in information technologies and 15 years of experience in remote sensing. She is a Senior Scientist at EOSDA responsible for developing technologies for satellite monitoring and surface feature change detection. Kateryna is an author of over 60 scientific publications.
Recent articles
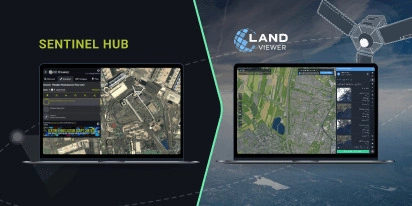
Transition Guide From Sentinel Hub EO Browser To EOSDA LandViewer
Need an EO Browser alternative that’s just as familiar but more flexible? EOSDA LandViewer offers free recent imagery, a vast satellite data archive, and advanced analytical tools.
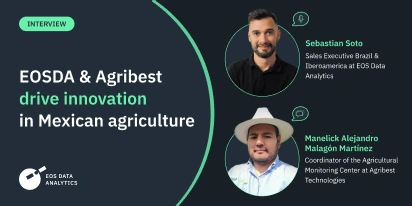
EOSDA & Agribest: Driving Agtech Growth In Mexico
In this insightful interview, Agribest shares how its collaboration with EOSDA is shaping Mexico’s agricultural future, with a focus on profitability, sustainability, and technology.
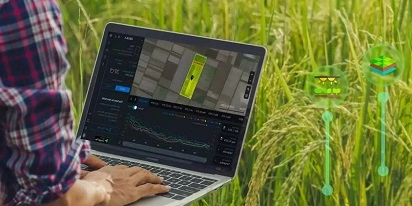
Top Ready-Made Agriculture Tools & Softwares for 2025
Agriculture softwares are silent partners in the field, working tirelessly behind the scenes to support farmers with insights and precision to cultivate sustainable agricultural ecosystems.