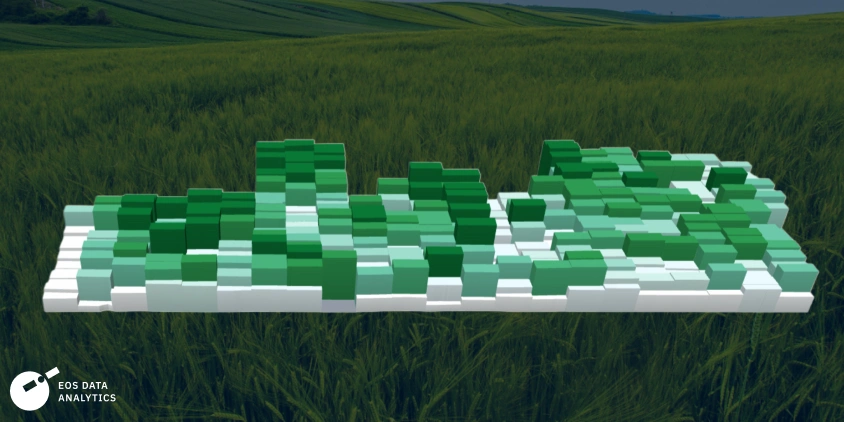
EOSDA Enhanced PySawit Model To Improve Its Accuracy
Oil palms that usually grow in the Asia-Pacific region are now facing more and more dangers due to climate change. Drastic weather events cause yield drops and make the growers wonder — is there an end to it? What should they do next, overcome the conditions, or give up? Of course, the first option makes much more sense.
The first thing that comes to mind is to study the problem, i.e., to get a better understanding of the impact of climate change on palm oil yields. Growers need reliable yield prediction tools for that. Modern tech already allows producers to gather historical data and get insights on what to expect in the upcoming season. EOS Data Analytics, a global provider of AI-powered satellite imagery analytics, offers one such solution — helping oil palm growers predict their yields accurately.
Oil Palm Market
The oil palm market is now large and continues to grow. The whole market size in 2023 accounted for $68 billion, where the Asia-Pacific region generated more than 73% of the revenue share in 2023 . In 2024, that number only grew, driven by increasing demand for palm oil in the food, cosmetics, and biofuel industries . Out of the countries in the region, Indonesia is the largest palm oil producer not only there but in the world, using it in biofuel production and trying its best to lessen the environmental impact of the industry .
The growth perspectives are promising, with the prediction of rising by $57 billion between 2024 and 2028 . Precedence Research says that the oil palm market is expected to reach around $121.80 billion by 2034, growing at a CAGR of 5.4% from 2024 to 2034. The numbers are astonishing, and more players are entering the market to reap those beautiful gains. No wonder producers constantly look for new ways to bring them a competitive edge. Yet whatever such ways may be, their profitability must be estimated before changing the palm tree growing process. This is where a yield prediction tool would come in most handy.
As a result, at EOS Data Analytics, we have observed yield predictions have become a critical requirement for various stakeholders in the palm oil industry. Public and non-governmental organizations rely on accurate yield forecasts to monitor production trends, assess sustainability efforts, and inform policy decisions to minimize environmental impact. Similarly, companies that have undergone IPOs depend on precise yield data to enhance their business monitoring and reporting practices. These predictions are needed when providing transparency to shareholders, meeting compliance obligations, and demonstrating the company’s commitment to sustainable and efficient operations, particularly in a sector that faces scrutiny over its environmental and social footprint.
Manual Methods For Oil Palms Yield Prediction
Several methods can be used to predict yields. Historically, the factors considered in yield prediction were palm age, soil type, leaf and soil testing, and palm growth parameters. Let’s look at them closer. The age of palm trees influences yield and bunch production rates. The soil type impacts palm growth, health, and fruit development. Leaf and soil testing is a method for nutrient assessment. And palm growth parameters include physical measurements that correlate with yield potential. The techniques used were counting black bunch, ripe and unripe fruit bunch, and cluster, as well as age-based estimation, all of which are done manually, one by one, of course.
However, those methods are increasingly challenging to follow. High dependency on labor and potential people shortages, slow data collection that delays decisions, and inaccurate and inconsistent manual records – these are the reasons palm oil growers look for new, more technological, and automated ways of calculating yield prediction.
When I spoke with clients in Malaysia, they emphasized a major challenge with labor shortages. It’s becoming harder to find people willing to work in the field because many now prefer the comfort of office jobs over physically demanding work. This challenge is also evident in Indonesia, where similar trends are emerging. Manual fieldwork is not only time-consuming but also highly demanding, particularly for large-scale oil palm producers managing hundreds of thousands of hectares.
The manual data records are also unreliable, as the data often gets twisted, replaced, and eventually lost. However, there is a way to use biophysics and calculate everything automatically instead of manually using data modeling.
New And More Accurate Yield Prediction Model
The PySawit model is an enhanced biophysics-driven approach to oil palm yield prediction. It utilizes biophysical relations and is driven by weather information, soil properties, planting density, water and temperature stress, and other parameters to predict yield. The original model components include meteorological, crop, soil moisture, energy balance, and photosynthesis data. That data is used for different functions of the model:
- Meteorological data is used to update information for the current day and calculate secondary parameters essential for modeling.
- Crop data is based on simulations of the growth patterns of palm trees and accounts for maintenance activities such as pruning and fertilization.
- Soil moisture data allows the calculation of layered soil moisture, assessment of water stress levels, and evaluation of the influence of groundwater on soil moisture.
- Energy balance helps calculate the canopy temperature to calculate the photosynthesis optimal conditions and analyze the balance between energy inputs and outputs that affect plant metabolism.
- Lastly, photosynthesis data is used for modeling the assimilation of CO₂ during photosynthesis and defining the further growth stages based on photosynthetic activity.
EOS Data Analytics decided to improve the PySawit model accuracy by up to 90% from the 78% it had already allowed. EOSDA’s science team added additional components and then recalibrated the updated model. They implemented a semi-automated approach, allowing adjustments based on parameters such as palm variety and improving accuracy and variability.
The updated model takes advantage of the higher-resolution weather data sources to offer improved precision in climate predictions. Vegetation index data derived from satellite images also enhances model accuracy. Finally, soil moisture indicators help reveal plant water stress more precisely if compared to the original model’s capabilities.
Feature | Enhanced PySawit Model | Manual Approach |
---|---|---|
Accuracy | Achieves up to 90% precision through automated modeling. | Depends on labor quality; prone to human error. |
Update Frequency | Offers bi-weekly, monthly, and quarterly updates via automation. | Irregular; tied to field worker availability. |
Labor Dependency | Reduces the need for extensive manual work, addressing shortages. | Requires significant manpower; vulnerable to shortages. |
Data Integration | Combines satellite imagery with environmental data for monitoring. | Limited to manual observations without satellite input. |
Environmental Monitoring | Tracks 5+ weather parameters, soil data, historical yields, and canopy temperature. | Focuses on palm age, soil type, and basic growth parameters. |
Approach | Leverages biophysical predictors like LAI, soil moisture, canopy temperature, and CO₂ rates. | Relies on physical observation and manual bunch counting. |
As more and more parameters are included in the model, it becomes more precise. To illustrate, if the initial PySawit used weather data of about 9 km or 5 km spatial resolution, our team used weather provider data with a 90 m spatial resolution. This allowed us to get the data for every specific plantation and not the whole plantation block or region.
It is important to add weather information to the model because there is a peculiarity of the region — farmers may reseed crops due to adverse weather. Thus, for the model’s accuracy, it was necessary to know both the reseeding date and the weather conditions on the plot.
Additional parameters and input data may impact the model’s accuracy. However, each project is unique and brings its peculiarities into the calibration process, so there can be no all-in-one solution for the ultimate model update.
Parameters Used To Calibrate The Model
Project implementation entails several datasets. The essential data comprises age and replanting rates, planting density, thinning strategy, area of interest (AOI) contours, and historical yield. The planting density also includes the total number of trees per hectare. The thinning strategy should be accounted for if ever used. AOI contours embody vector format, the number of stands, and hectares. Last but not least, the historical yield from the past 3-5 seasons is needed, including monthly, quarterly, or yearly data.
On the other hand, additional data is not as essential, but it will allow the model to be calibrated even further. Here, soil maps and composition, groundwater table depth, average weight per palm, data from meteorological stations or soil sensors, and any crop management data, including fertilization, irrigation, and tillage, will suffice.
Using our enhanced PySawit model has several benefits. Its enhanced accuracy leads to improved financial planning, sustainability support, optimized harvest planning, risk management, market competitiveness, cost reduction, and resource allocation optimization. Flexible modeling frequency allows for data-driven decision-making, time savings, and accurate supply chain management.
Model In Action: How It Can Be Used
One of the most prominent clients taking advantage of our enhanced PySawit model is based in Indonesia. They manage an estate of about 15-17 thousand hectares and wanted to know the expected yield of season 2024-2025. Here’s what the model output looks like – specifically, the vector layer of predicted yields on different parts of the field.
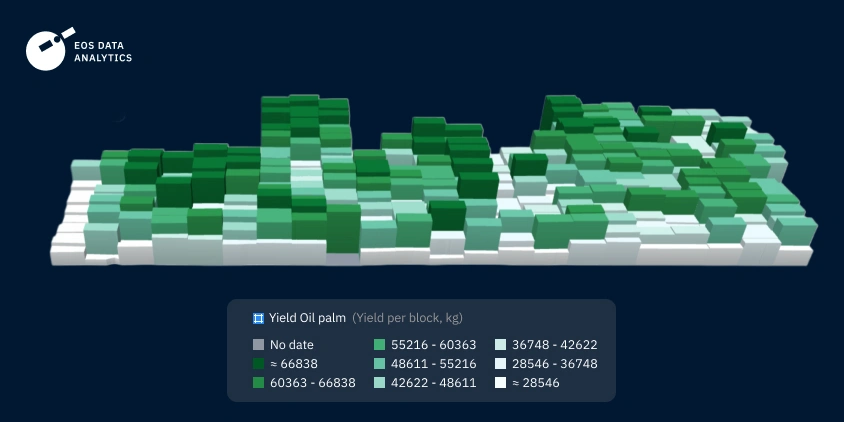
Experienced farmers used to traditional farming methods are often wary of new technologies, as their benefits are not always obvious, and the results are not always reliable. However, despite the complexity of the PySawit model itself, its use is as simple as possible — in the end, you get data on the predicted yield in a form similar to the one you got before with manual calculations, but the accuracy will be higher.
The world moves on, and so should the crop growers. Not long ago, people didn’t know what the internet was, but now, we use it in every aspect of our lives. The same goes for precision agriculture and its methods — one day, farmers will not even realize there was a time when people counted their palm trees by hand and calculated their yields this way.
Our team is flexible and ready to take on any challenge that comes our way.
We are ready to twist the model based on your inputs. We want to improve accuracy by making several iterations and prove that PySawit can be much more effective than any model you are using now.
If you’re considering changing the approach to growing oil palms, let’s talk. Our experts, like Sergii and Oleksii, can help you determine what data is required for the project. With the growing market demand and toughening weather conditions, it is smart to look into the future and make informed decisions. EOSDA is here to lend a hand. Let’s grow and change together.
About the author:
Kseniia Kunakh has over 6 years of writing experience, working in various domains, including business, educational, and media-directed texts. Kseniia’s previous experiences as a development manager in a Ukrainian eco-NGO and as a talent matcher in an IT company make her a perfect combination of someone who is passionate about eco-tech innovations and can communicate about them with ease.
Recent articles
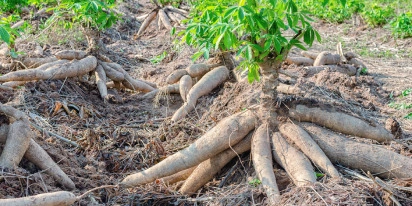
Growing Cassava: From Land Choice & Planting To Harvest
Growing cassava can work well even in poor soils and dry conditions, unsuitable for most other crops. So, farmers can achieve high yields even under less-than-ideal conditions without too much input.
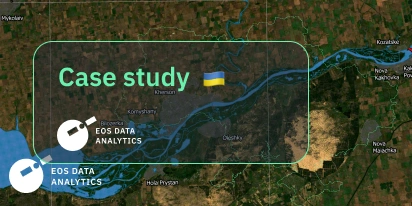
Kakhovka Dam Flooding Detection In Ukraine For PEJ
EOSDA helped Project Expedite Justice calculate the flooding of the surrounding area around the Kakhovka Dam destruction on June 6, 2023, for the justice report for the International Criminal Court.
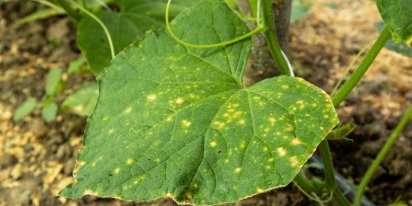
Downy Mildew: How To Identify, Prevent, And Control
Controlling downy mildew — an aggressive crop disease — requires early identification and action. Targeted prevention strategies have a direct impact on the overall economic sustainability of farming.