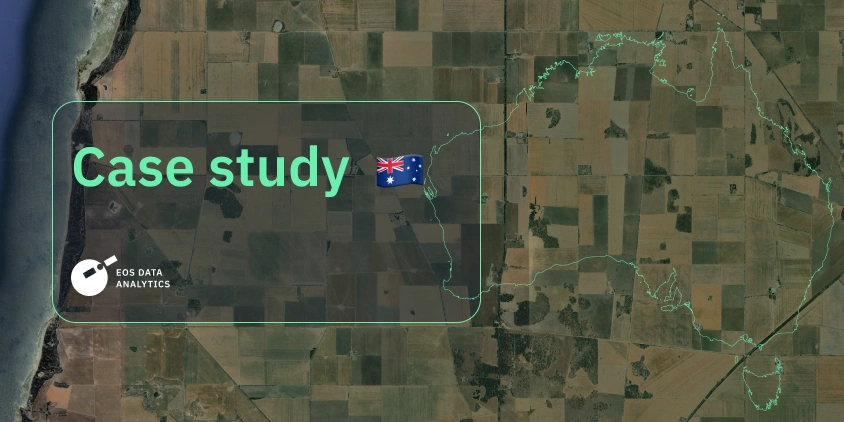
EOSDA Classified Crops In Australia For Climate Reports
One innovative climate analytics company in Australia offers a scalable solution for businesses serving the land and agricultural sectors. To meet the requirements of a proof-of-concept project for one of their clients, they needed precise data on crop types and field boundaries of an area in the York Peninsula.
Tight deadlines and a lack of ground-truth data made satellite data analytics the only way to generate accurate insights. Within only six weeks, EOS Data Analytics provided the company with the data necessary to fulfill the expectations of its clients.
Challenge | A company required accurate crop classification and field boundary detection for a proof-of-concept project in South Australia’s Yorke Peninsula but lacked reliable ground-truth data for model training. |
Solution | EOS Data Analytics finetuned and applied its field boundary detection and crop classification solutions to analyze Sentinel-2 satellite imagery and provided outputs in six weeks. |
Outcome | The client successfully demonstrated the proof-of-concept to their end user. |
Overview: Climate Reporting Needs Accurate Data
One Australian company is focused on advancing climate reporting and emissions management. It develops innovative tools to measure and manage greenhouse gas emissions. These tools are specifically tailored for the agriculture and land use sectors.
This company aims to provide its clients with precise, compliant data to meet growing regulatory demands. Their stated mission is to enable effective climate risk assessment and encourage actionable environmental responsibility across industries.
Challenge: Tight Deadlines, Data Gaps, Similar Crops
The company operates a climate analytics platform designed to help organizations monitor their greenhouse gas emissions. Within this platform, users can also calculate such emissions from agricultural activities.
However, such calculations require accurate input data about land use, such as field boundaries and land cover types. Often, obtaining such data is challenging since ground-truth information – i.e., field-level data validated by direct observation – can be unavailable or prohibitively expensive.
In South Australia’s Yorke Peninsula, the company decided to conduct a proof-of-concept project and needed to address this issue precisely. The methods for land cover classification and boundary detection they had were not accurate enough to meet the need for detailed, region-specific information.
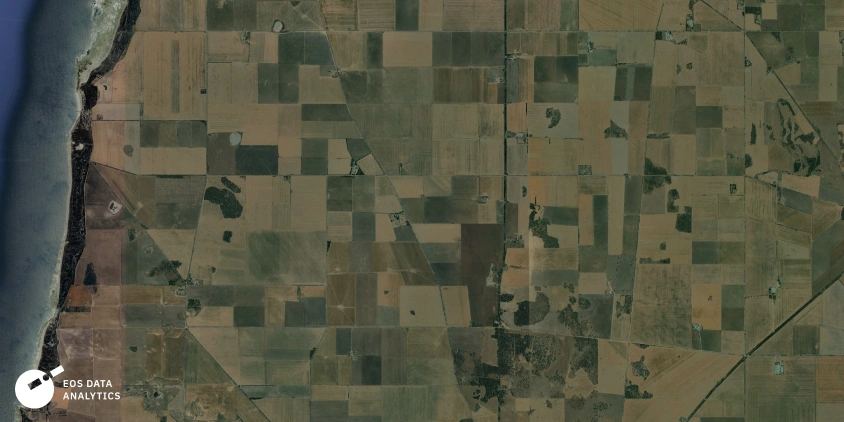
In this case, the company’s client was also expecting a custom proof-of-concept on the land cover classification possibilities within a tight four-to-six-week timeline.
Adding to the complexity, South Australia’s diverse agricultural landscape made it difficult to distinguish between certain crops, such as wheat and barley. This required advanced modeling approaches and high-quality data that the company could not independently procure in the available timeframe.
So it sought a scalable, efficient solution to detect field boundaries and classify land cover accurately. Success would mean not only meeting the immediate client’s expectations but also unlocking broader opportunities in other regions and industries.
Solution: From Sentinel-2 Imagery To Accurate Land Cover Maps
To meet the company’s need for accurate land cover classification, EOS Data Analytics utilized its field boundaries detection and crop classification solutions in combination with the Sentinel-2 satellite imagery, known for its 10-meter resolution and availability at no cost.
We have a robust land cover classification solution at EOSDA, but for this case, the specific requirements demanded a higher level of detail. That’s why we opted for our crop classification solution — it provides much finer granularity, which was essential for distinguishing between target crops in the region.
The project, conducted between late August and late September 2024, followed a structured, multi-stage approach:
1. Vegetation and regional analysis: The team analyzed the agricultural characteristics of the Yorke Peninsula during the 2023 growing season.
2. Satellite data acquisition and preprocessing: Sentinel-2 images were selected based on a cloud coverage threshold of 30–40%. The data then underwent atmospheric corrections and other preprocessing steps to make it ready for analysis.
3. Boundary detection. Field boundaries were detected using EOSDA’s field boundary detection solution and manually refined to perfect accuracy.
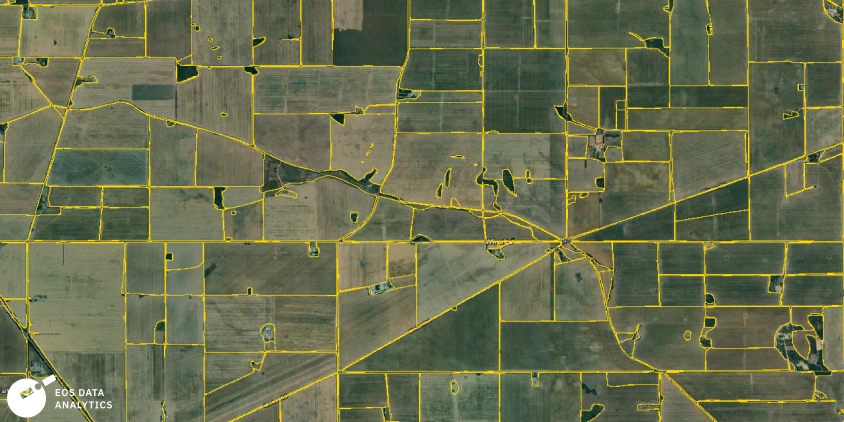
4. Model adaptation and training: EOS Data Analytics used the Conv-LSTM model for crop classification.
Initially, a model trained on datasets from Europe was used to apply the crop classification solution and get the first results. Meanwhile, publicly available datasets from nearby regions in South Australia allowed the creation of an even better-calibrated model.
5. Crop classification: The classification process identified key crops, including wheat, barley, oats, and canola, within a defined area of interest of over 3 thousand square kilometers.
While distinguishing wheat from barley posed a challenge due to spectral similarities, these crops were grouped under a “cereals” class for the proof-of-concept phase. With access to ground-truth data and additional model training in future iterations, we anticipate achieving successful differentiation, as demonstrated in Europe, where most extensive datasets and multiple training cycles yield highly accurate results.
6. Validation and refinement: Detected field boundaries were reviewed and corrected where anomalies, such as small erroneous polygons, were found. This step ensured the outputs were consistent and usable for the company’s needs.
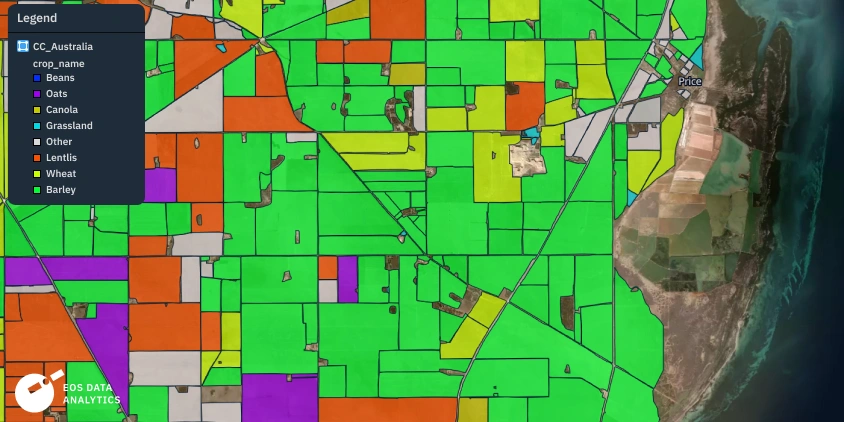
7. Reporting and deliverables: The final deliverables consisted of vector layers with classified crops and field boundaries designed for seamless integration into the company’s tools.
The project team at EOS Data Analytics included two GIS analysts, a data engineer, a sales lead, and a business analyst, which helped combine expertise in geospatial analysis, machine learning, and project coordination.
Outcome: Actionable Results Within Weeks
The EOSDA team successfully delivered detailed crop classification and field boundary layer for the defined area of interest in South Australia’s Yorke Peninsula. The company received high-quality data for the proof-of-concept solution they later presented to their client.
The classification outputs met the company’s needs despite challenges such as the lack of ground-truth data and tight deadlines. These results aligned well enough with available official statistics, which confirmed the credibility of the deliverables.
The project’s success established the scalability of EOSDA’s crop classification and field boundary detection services, and the company is now better positioned to offer region-specific insights to its clients.
This collaboration showcases how satellite data analytics can overcome data scarcity and enable organizations to deliver insightful data even in challenging contexts.
About the author:
Maksym Sushchuk is at the forefront of realizing EOSDA's vision to make space tech a global driver of sustainability on Earth. He has over 15 years of experience in journalism and content creation for prominent Ukrainian startups, charitable funds and ESG businesses. As Head and Co-founder of PR Army Maxim brings attention to the human and social tolls of the aggression against Ukraine.
Recent articles
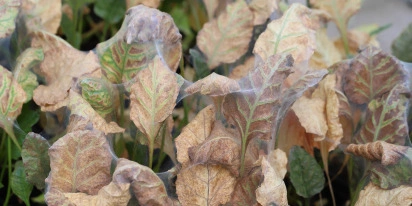
Spider Mites: How To Identify And Get Rid Of Them
Spider mites can rapidly overwhelm crops without proper monitoring. This guide covers essential aspects of pest management in commercial agricultural settings.
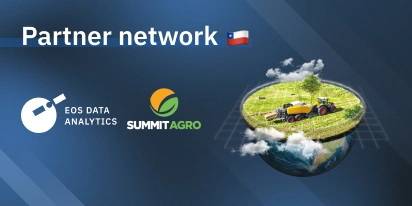
Summit Agro Chile Partners With EOS Data Analytics
EOSDA announces a partnership with a Chilean-based international input seller, Summit Agro, to visualize vegetative changes in local crops in connection with climatic and environmental conditions.
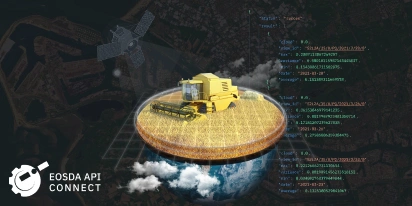
How EOSDA API Connect Helps Businesses Shape The Future
Antonio Quesada, Customer Success Manager, discussed EOSDA API Connect and its endless customization possibilities for the clients, diving into customer success stories and explaining API with ease.