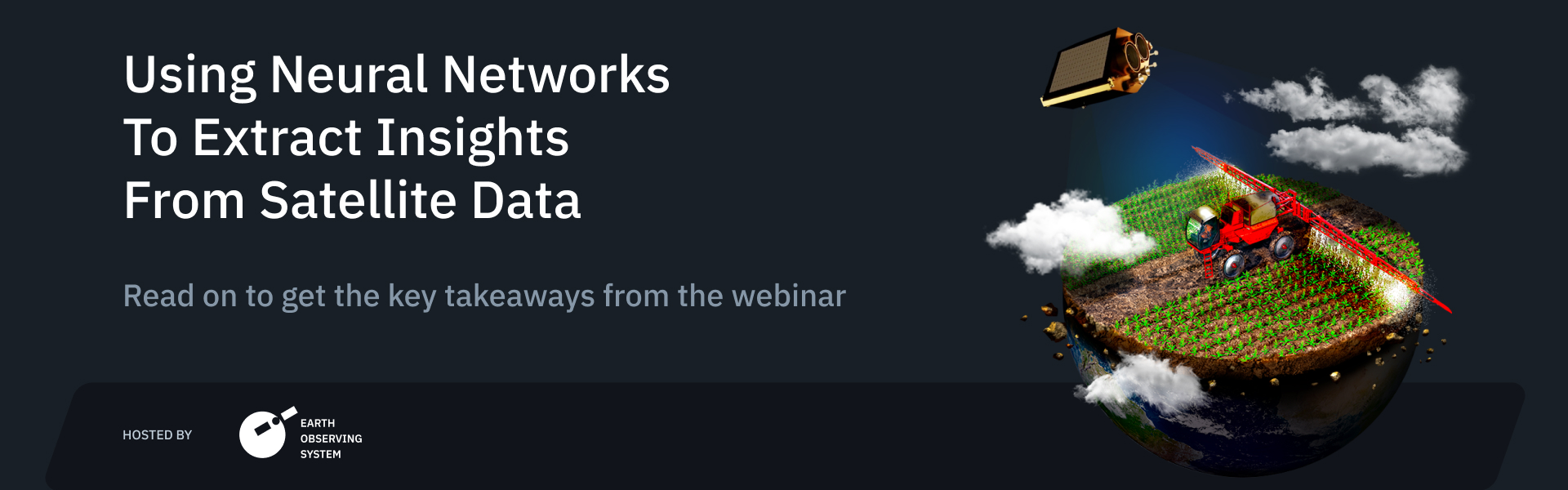
Using Neural Networks To Extract Insights From Satellite Data
On March 29, EOS Data Analytics (EOSDA), a global provider of AI-powered satellite imagery analytics, held a webinar about the capabilities of neural networks in solving classification and prediction tasks for agricultural and forestry industry users.
The webinar presenter Lina Yarysh, Director of Customer Success at EOS Data Analytics, explained how neural networks, deep learning algorithms great at pattern detection, recognize what is depicted in images.
The host told what agriculture and forestry-related tasks neural networks are great at solving — classification of land cover, crop and tree types, cloud masking, and detection of deforestation areas. The specialists also shared two crop classification use cases.
You can rewatch the webinar recording and review the presentation.
The event targeted managers and tech specialists from agricultural (including food production) and forestry companies and those having clients from these industries. The webinar gathered participants from 15+ countries worldwide, including India, Germany, Italy, Brasil, Bolivia, the Philippines, etc.
The Food and Agriculture Organization (FAO) estimates that by 2050 we will need to produce 60 percent more food to feed 9.3 billion people. Ag businesses that must meet food transparency demand are already struggling to keep their agricultural footprint minimal. FAO also notes that science and technology are the main drivers of agricultural productivity. And it’s true. AgTech companies have been developing solutions that allow farmers to get quick, near-real-time insights for optimizing their farm management strategies. With cutting edge solutions, farmers can improve yields, decrease waste and loss of yield, cut operating costs while staying sustainable.
EOSDA continues investing and developing scalable software to help end-users meet their goals by making daily data-based decisions.
Proprietary Solutions By EOS Data Analytics And Their Capabilities In Brief
Satellite imagery analytics tools by EOSDA support decision-making for users from 22 industries, notably agriculture and forestry (farmers, food traders, input suppliers, agriculture insurance and financial companies). EOSDA Crop Monitoring and EOSDA Forest Monitoring allow for evaluating the condition of crops, forests, and soil and making predictions about observed areas by analyzing satellite images and acting as sources of current and historical weather data.
Our solutions are built in-house: we have a team of researchers, product managers, data analysts, data scientists, GIS specialists, software developers, QA testers, and other professionals working together. It makes us independent from contractors and allows keeping complete control of product development. And since the beginning of our work, we chose machine learning as a method of data analysis.
Deep Learning With Artificial Neural Networks. How We Train Neural Networks And What Tasks Solve With Ease
Machine learning is a data science discipline that aims to enable machines to learn from data and extract insights without being explicitly programmed. These algorithms are excellent in analyzing and interpreting vast amounts of data.
Deep learning is a subfield of machine learning that uses complex (deep learning) algorithms with neural network architectures — artificial neural networks (ANNs). ANNs are very powerful at image recognition (classification). Convolutional Neural Networks, one of the most popular deep learning algorithms, are used in EOS Data Analytics products.
To enable neural networks to recognize specific objects, data scientists train them with large datasets of images with examples of these objects, where each image is labeled (annotated) with a category to which it belongs: a cat or a dog, water or a land cover, etc.
Thanks to neural networks’ ability to identify patterns, they solve various classification and prediction problems for EOSDA customers, such as land cover and crop type classification based on information about the health of crops and soil conditions extracted from satellite images.
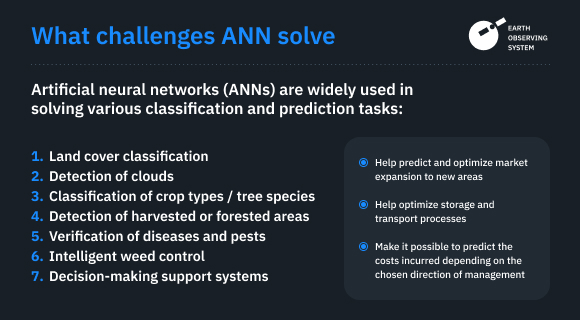
During the final part of the webinar, the presenter explained how the EOSDA professionals solved real-life problems: classified crop types in Ukraine and sugarcane in Brazil and detected deforested areas with tree type classification.
Classification Of Crop Types Within The Governmental Land Transparency Program In Ukraine
The multi-year project with the World Bank — agricultural land classification in Ukraine — began in 2016. The EOS Data Analytics assignment was to classify agrarian lands all over the country, digitize field borders, and classify the type of crops (up to 15) growing on each field. The total area of arable land for processing was 41 million ha.
Numerous agriculture sector parties benefit from crop classification data:
- Farmers: managing daily activities, estimating potential yields, using crop rotation data, or comparing year-to-year results of their work.
- Traders: setting price points and for ecological considerations such as nutrient inputs.
- Insurance companies: supporting reimbursement decisions with data.
- Government entities: developing and managing agro databases, negotiating compensations with farmers.
For the ground-truth data collection, specialists were making trips twice a year, during summer and winter, to map crops that grow during these seasons. Every year, agronomists were gathering thousands of data points to keep crop maps relevant. Data scientists, in turn, have been training deep learning algorithms with fresh images to increase crop classification accuracy gradually. As a result, the highest accuracy (more than 90%) was achieved for recognizing such crops as maize, sunflower, and soya.
As a result, EOSDA specialists digitized all cultivated lands, having not only classified more than 15 crops (e.g., wheat, sunflower, maize, alfalfa, sugar beet, barley) but also other land cover classes (e.g., forest, grassland, waterland). They also prepared annual field-scale crop maps for six years, from 2016 to 2021.
Sugarcane Classification In Brazil: Detection of Sugarcane Mills And Area Estimation
Within the project, specialists had to solve two problems: detect sugarcane mills and harvested stacks of sugar cane (this crop is harvested several times a year) on a given date.
Sugarcane mills classification. The EOS Data Analytics team has collected ground truth data covering 1,000,000 hectares. Images were taken with Sentinel-1, Sentinel-2, and MODIS-Terra satellites in 10 countries and labeled for further model training.
Specialists compared the effectiveness of two models and managed to get the highest percentage of accuracy (over 94%) with a Conv-LSTM model, which combines the benefits of convolutional (CNN) and long short-term memory (LSTM) networks and allows for analyzing spatial time series data.
Harvested field index (status) detection. The task was to define a ratio between harvested area and the total area of the field.
The EOSDA team has successfully detected the harvesting status for 6500 fields with a total area of 47,000 ha. Data scientists provided reports on the harvesting status every 10 days.
The last case the presenter talked about was the detection of areas covered with forest and deforested areas in Tasmania. The specialists solved the problem by using an ANN to compare two cloudless images taken in given months, quarters, or years.
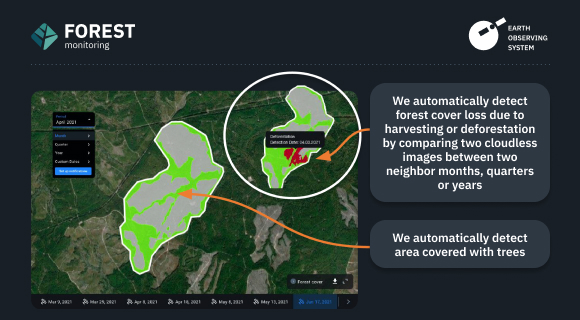
I’m happy to have engaged with agriculture and forestry businesses specialists. EOS Data Analytics strives to continue contributing to the growth of the sectors by popularizing precision agriculture methods via its AI-based satellite imagery analytics platforms and custom solutions.