Crop Type Classification Using Remote Sensing
Classification of field crops is an essential part of agriculture as a business. Identifying crops using traditional methods can be quite a daunting task.
EOSDA offers a faster and much easier way of solving this problem relying on years of experience in precision agriculture, as well as expertise in the application of AI-powered algorithms, and remote sensing.
By combining Synthetic Aperture Radar (SAR) data with optical imagery, we can assign a class to each type of crop recognized by a trained neural network in any area of interest and build a crop type map suitable to the customer’s needs.
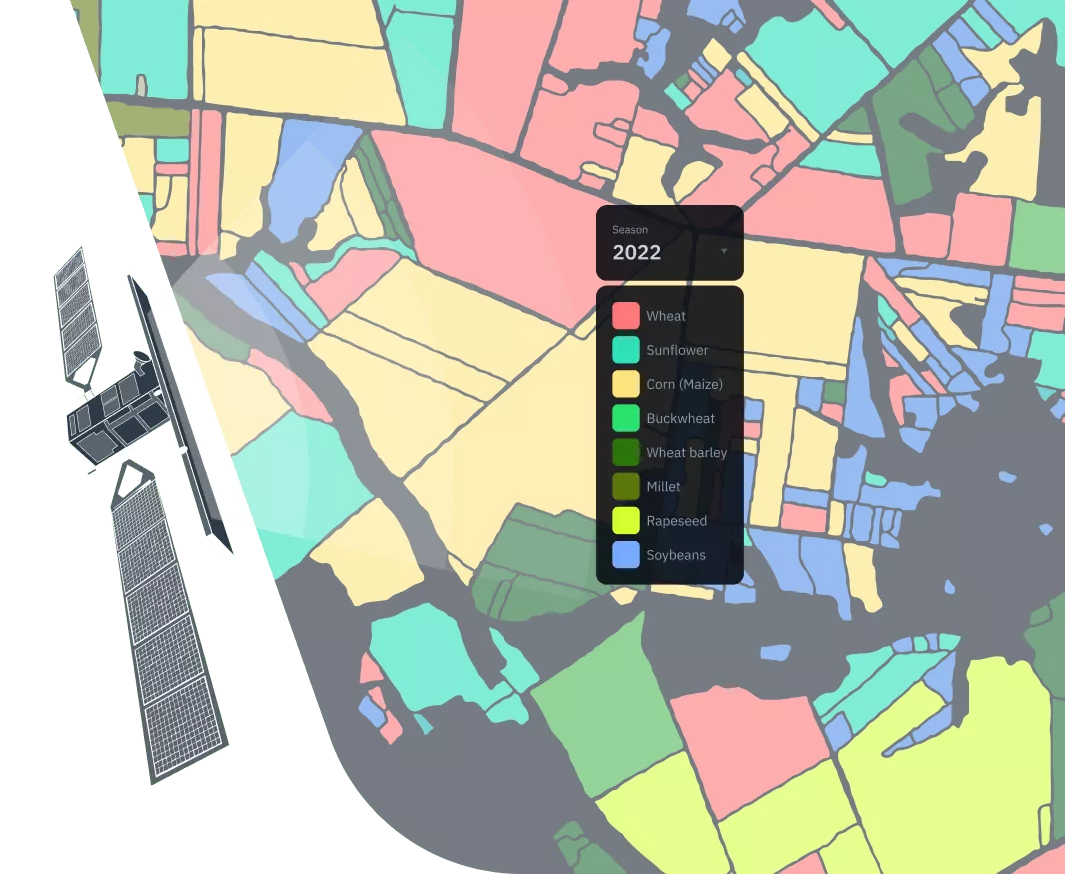
Crop Classification Solution In Numbers
Crop type maps with an accuracy of up to 90%, depending on the completeness of ground data and availability of regular satellite scenes.
Get cropland masks at a 10-m resolution in .geotiff or .shp formats.
Crops identified for any area, even as small as 3 ha.
Our algorithm identifies crops almost anywhere on Earth.
If conditions are favorable, our qualified RnD team requires only several weeks to complete research and deliver an accurate crop classification map to you.
Our trained neural networks can classify over 15 different types of crops.
Watch video about our crop classification solution
It is possible to improve global food security by modifying food management practices and making them more sustainable. That is why EOSDA developed a remote crop classification solution by fusing satellite data analytics with the latest AI technologies that allows large scale agro producers to increase profits in a more environment-friendly way.
What Crop Classification Achieves
- Taking inventories for large areas and estimating yields.
- Keeping extensive crop rotation records for selected areas.
- More transparent crop type data for the validation of compensation requests.
- Management of land use is much easier with crop type data.
- Crop identification allows traders to set price points on the market.
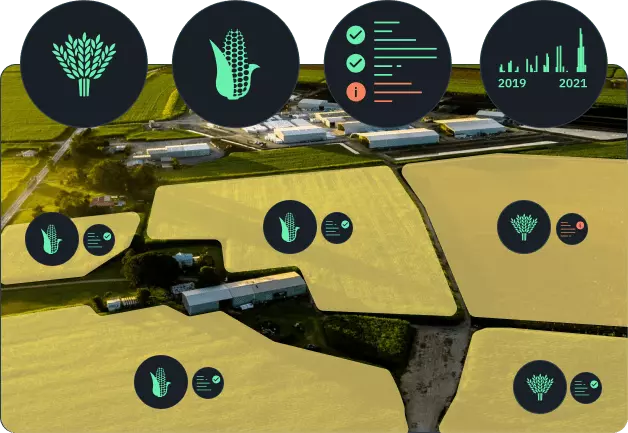
Advantages Of Our Approach
Data obtained only from the optical satellite imagery may be incomplete or non-existent due to cloud coverage, fog, etc, making crop identification difficult or impossible.
Synthetic Aperture Radar (SAR) is an active sensor emitting microwave radiation. As a result, it does not require the reflected sunlight to collect data from an area of interest. By combining SAR data with optical imagery, we easily solve the cloud coverage problem. In fact, this allows us to classify crops in an image that is taken in poor visibility or even at night.
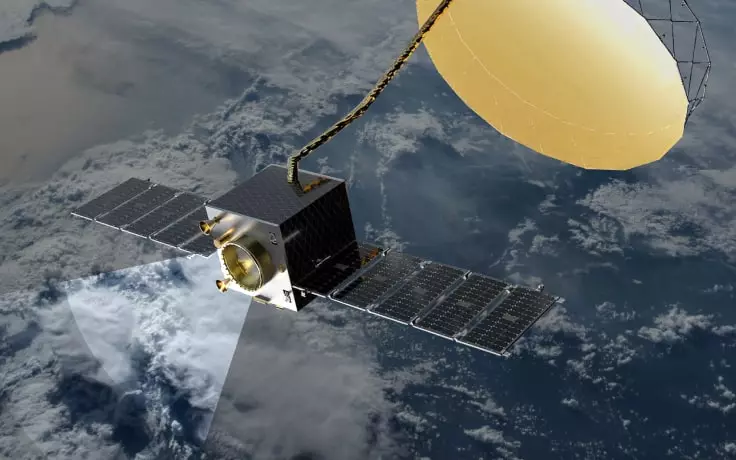
Relying on Sentinel-2 time series of multispectral images means there’s quite a lot of data to process even for one tiny field.
Our arsenal of pre-trained models can handle almost any request. For any new area or crop type, we can easily adjust the existing neural network models and quickly obtain results.
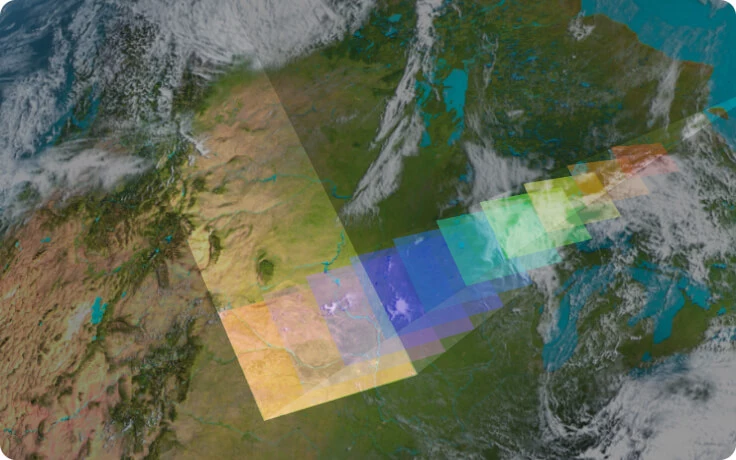
Classification of crops over vast areas is more problematic because each satellite can only capture a limited area at a time.
Thanks to the pixel-based segmentation performed by our deep-learning algorithms, we can obtain more data in a shorter period of time. Crop classification can also be carried out much faster if we receive complete and accurate ground-truth data for the region of interest. In 2023, EOSDA launched its own satellite (EOS SAT-1), which significantly reduced the final delivery rate of our custom solutions.
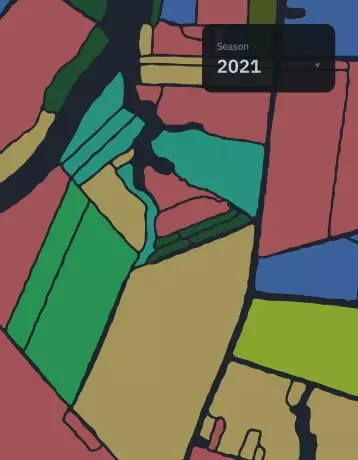
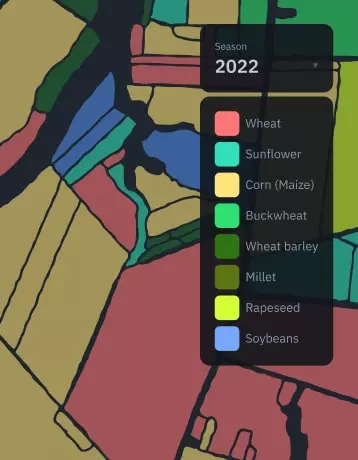