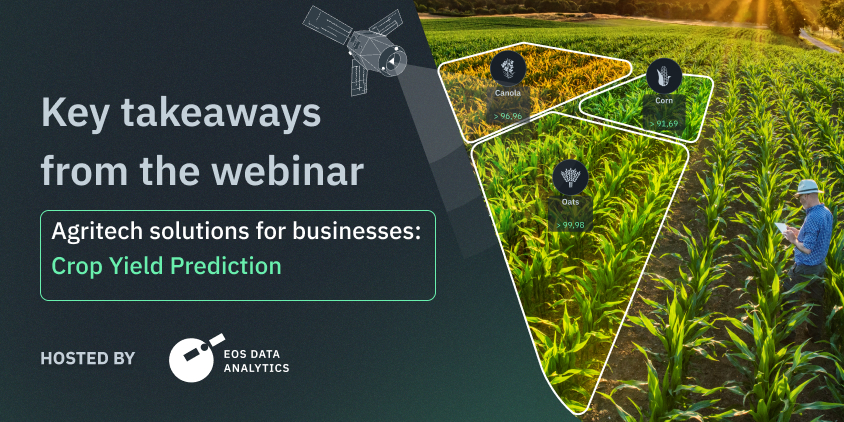
Agritech Solutions For Businesses: Crop Yield Prediction
On June 29, 2023, EOS Data Analytics, a global provider of AI-powered satellite imagery analytics, held a free webinar dedicated to the exploration of crop yield prediction, EOSDA’s custom agritech solution used by agribusinesses around the world.
On the webinar, such topics as yield prediction approaches, their requirements, and expected results, as well as the success stories of EOSDA’s clients who leveraged the company’s crop yield prediction solution were covered.
To access the presentation, follow the link.
Various representatives of agritech developers, insurance providers, input suppliers, food producers, government entities, as well as investment funds and educational organizations, attended this online event.
The following speakers had been uncovering the subject during the webinar:
- Yevhenii Marchenko, Account Executive at EOSDA
- Aleksey Neskorovnyi, Business Analyst at EOSDA
The webinar started with an introduction to EOS Data Analytics, a comprehensive overview of its inception, core values, and the innovative solutions it offers to the agricultural industry.
Aleksey Neskorovnyi started his part by explaining the concept of crop yield prediction. This process involves estimating the potential yield of a specific crop within a defined area for a particular growing season. To derive these estimates, diverse sets of data, including weather patterns, soil properties, crop genetics, management practices, and statistical data are required to be presented. Then, a sophisticated multi-tiered algorithm to estimate the yield at any scale is employed.
The benefits of yield prediction extend to a wide array of stakeholders, including producers, input suppliers, food production companies, government food security departments, trading companies, banks, insurance companies, and international organizations such as the World Bank and the United Nations.
Aleksey further elaborated on the two primary approaches to crop yield prediction. The first is the statistical yield prediction model, which relies heavily on historical datasets, primarily past crop yields. The second is the biophysical yield prediction model, which is based on the biophysical parameters of the crop and its phenological characteristics, such as variety, growth stages, water demand, optimal temperature, and humidity, among others.
The statistical yield prediction model is applicable when there is an ample dataset for training, primarily historical yield data. On the other hand, the biophysical yield prediction model can be utilized when historical yield data is not available. This model can be applied multiple times per season, providing more comprehensive insights.
To provide even more accurate results using the last mentioned model, initial calibration is crucial. The Leaf Area Index (LAI) assimilation allows for achieving an impressive accuracy of up to 95% in 30% of the fields, a significant improvement over traditional methods (for instance, the WOFOST approach).
Then, the speakers listed the types of input data required for the model to generate the most accurate results and revealed what the output looks like and the various formats in which it can be provided.
Yevhenii Marchenko and Aleksey Neskorovnyi then showcased several of EOSDA’s yield prediction use cases carried out in Ukraine and other countries, focusing on crops such as corn, winter wheat, and sunflower. The accuracy of these predictions – made both on regional and field levels – was approximately 75-80% two months before harvest, and up to 80-95% two weeks before harvest, depending on the crop.
To conclude the webinar, the speakers revealed more details about EOS SAT-1, a multi-purpose satellite, focused on agriculture, forestry, natural disaster management, and urban monitoring. This independent satellite, launched in 2023, provides high-quality imagery, further enhancing the accuracy of the crop yield prediction model and offering numerous benefits to the agricultural industry.